1 Introduction
The four documented industrial revolutions represent key milestones in human history and have profoundly transformed the way we produce, consume, interact, and educate ourselves. According to Lee et al. (2018), the first industrial revolution was characterized by the introduction of the steam engine and the mechanization of production; the second focused on mass production and electrification of industry; the third brought about digitization and automation of production, and the fourth industrial revolution, or Industry 4.0, centers on the integration of emerging technologies such as artificial intelligence, robotics, and the Internet of Things into production and business management processes (Patiño; Ramírez-Montoya; Buenestado-Fernández, 2023).
According to Chituc (2021), in this context, the term “Education 4.0” arises, which is used to describe the transformation of educational systems in response to the fourth industrial revolution. Said transformation is due, in part, to the need to prepare students for the challenges of the future, including the increase in automation and digitization of the economy, as well as the demands of an increasingly competitive and changing labor market (Akimov et al., 2023).
In this regard, it is important to understand the challenges of learning in digital distance environments (Zapata-Ros, 2018). Specifically, Jurado Valencia (2016) emphasizes the weakness of pedagogical training among university professors and the excessive standardization of assessment as two of the most relevant factors contributing to the phenomenon of dropout rates during the first two years of Higher Education, which can be extended to the context of interaction in digital environments.
Taking the above into consideration, it is worth highlighting the various issues associated with the assessment of learning that have drawn attention in educational research in recent decades regarding these learning environments. In this sense, complex evaluative phenomena such as fraud (Martinez; Ramírez, 2017), information plagiarism (Chaika et al., 2023), identity impersonation (Pfeiffer et al., 2020), lack of self-assessment culture (Sanz-Benito et al., 2023), absence of learning visibility strategies beyond grade analytics (Cabra-Torres, 2010), and difficulty in making autonomous decisions by students (Gowin; Millman, 1981) are highlighted.
In addition to the above, authors such as Rodríguez (2013), Ruíz Martín (2020), Viñolas and Sepulveda (2022) and Wu and Gun (2021), and emphasize a weakness in the designs of learning assessment in terms of repetitiveness, monotony, or mechanization of assessment activities. Other authors, such as Mamani Choque et al. (2022), focus their attention on deficiencies in timely and effective feedback. Finally, another group of researchers, like Iafrancesco Villegas (2017), highlight the need to create much more empathetic assessment spaces where there is closer interaction between students and teachers, and the importance of learning styles is recognized.
On the other hand, in a general sense, it can be stated that the assessment of learning has been a topic of growing interest among the community of teachers and researchers in Education, as evidenced in Figure 1, which shows the number of articles related to learning assessment processes in the context of Distance and Technology-Enhanced Learning environments that were published in peer-reviewed journals indexed in Scopus. While this Figure demonstrates a growing trend parallel to the development and evolution of digital technologies, the low number of publications per year indicates that it is still a topic with ample room for educational research.
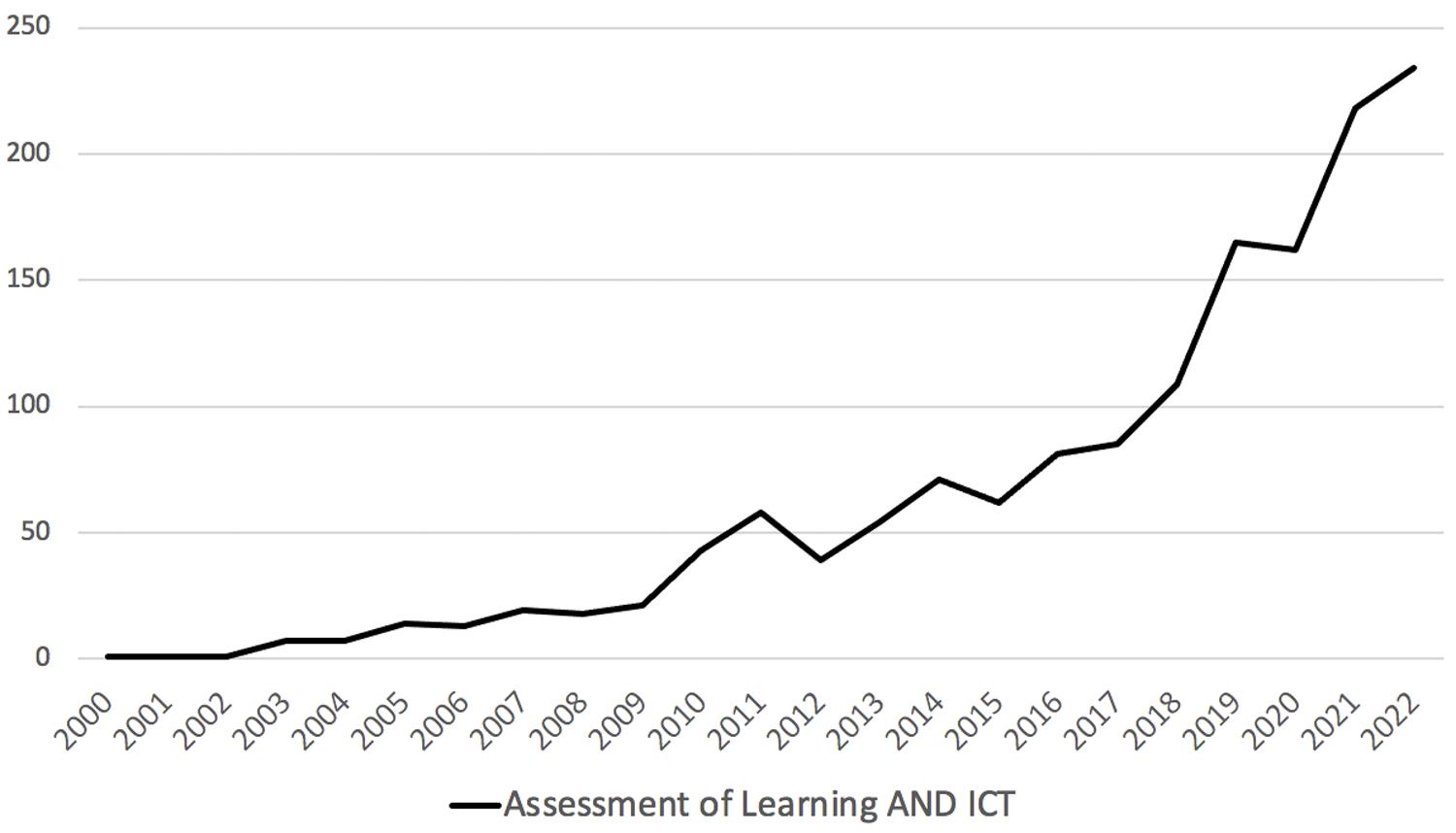
Source: Scopus (2024)
Figure 1 Peer-reviewed articles about the Assessment of learning and ICT published in Scopus-indexed journals
In this context, the need to effectively assess learning by implementing new approaches, logic, tools, and metrics in the evaluation process becomes particularly important.
1.1 Some brief insights on assessment in the era of artificial intelligence
Assessing learning in the era of artificial intelligence presents both opportunities and significant challenges, especially considering the capacity of artificial intelligence to transform how information is currently collected, analyzed, and utilized to assess student learning (Bitencourt; Silva; Xavier, 2022; Diyer; Achtaich; Najib, 2020; Parreira; Lehmann; Oliveira, 2021).
In general, experts in the field, such as Salazar, Ovalle and De La Prieta (2019) or Duque-Méndez, Tabares-Morales and Ovalle (2020), indicate that one key advantage of artificial intelligence in learning assessment is its ability to efficiently and accurately process large volumes of data. In this sense, machine learning algorithms designed to support assessment processes should have the capacity to analyze complex patterns in the data generated by students and provide valuable information about their performance and progress (Grimalt-Álvaro; Usart, 2024). This would assist educators not only in accurately reporting learning outcomes but also in making better-informed and more personalized decisions regarding individualized teaching and support for each student (Kaliwal; Deshpande, 2021).
However, there are also inherent challenges in AI-based learning assessment, such as ensuring the validity, reliability, and transparency of the results generated by automated assessment systems, as well as addressing ethical concerns related to the privacy of data collected and analyzed in these processes (Guan; Feng; Islam, 2023). Furthermore, finding an appropriate balance between the involvement of automated systems and human input in learning assessment processes is challenging. While artificial intelligence can provide valuable insights, we believe it should not completely replace the evaluation conducted by educators and experts in the field. In this regard, we endorse the views of Tataw (2023) and Burgess and Rowsell (2020) that learning assessment should be a holistic process that considers both quantitative and qualitative aspects of learning, including multiple variables and purposes.
Building upon the aforementioned, in this article, we aim to propose a perspective for approaching the creation of assessment support systems that utilize artificial intelligence, based on articulating three key concepts: cybernetics of self-regulation, homeostasis, and fuzzy logic.
1.2 Cybernetics of self-regulation and homeostasis: a response to cognitive imbalance
For a long time, a dichotomous relationship between the body and mind in human beings has been addressed, where although related, bodily processes have been treated differently from cognitive processes (Berent, 2023). However, as human beings are integrated entities, characterized by the intimate connection between bodily and mental functions, they cannot be separated, as they function together to shape our experience and existence (Bernier; Carlson; Whipple, 2010). For instance, bodily functions such as respiration, digestion, and movement are closely linked to our mental functions such as thinking, emotion, and perception (Trevarthen, 2012). In other words, our mental states influence our physical well-being, and vice versa; this interconnectedness reflects the complexity and holistic unity of the human being as an integral entity.
Taking the above into account, it is relevant to recall and explore the term “Homeostasis” and its application within the framework of this article. According to Kelkar (2021), Homeostasis is a fundamental principle in both biology and psychology, which describes the inherent tendency of living organisms to maintain a state of internal balance to self-regulate physiological and psychological variables and ensure optimal functioning.
When approached within the context of learning, homeostasis assumes a particularly interesting dimension, as it pertains to the equilibrium sought by the cognitive system to restore lost conceptual harmony due to the impact of cognitive imbalance generated by the learning process (Ciaunica et al., 2021). To understand this, it is important to recall that, according to Jean Piaget’s theory of cognitive development, cognitive disequilibrium is a state of conflict or discrepancy between existing cognitive structures and new experiences or information, which is a necessary condition for cognitive growth and development as it motivates individuals to adjust and reorganize their cognitive schemes to achieve a new equilibrium (Goswami; Chen; Dubrawski, 2020). This process of self-regulation allows students to harmoniously integrate new knowledge, connecting it with their existing knowledge base and constructing a deeper and more comprehensive understanding.
Now, having addressed homeostasis and cognitive disequilibrium, we will introduce the Cybernetics of Self-regulation to position this argument within the framework of digital systems, particularly in the context of using artificial intelligence. According to Mackenzie, Mezo and Francis (2012), cybernetics of self-regulation is a concept that refers to the process by which self-regulating systems, such as human beings (and now, certain developments in artificial intelligence), adjust their behavior in response to deviations between established goals and achieved outcomes. In the context of learning, Zachariou et al. (2023) and Prather et al. (2020), indicate that self-regulation involves students’ ability to monitor, regulate, and adjust their cognitive processes and behavior to achieve better performance, which is closely linked to metacognition.
1.3 So, what relationship emerges between homeostasis, cybernetics of self-regulation, and cognitive disequilibrium?
Up to this point, it has been posited that the learning process generates cognitive imbalances that need to be consistently resolved to consolidate the outcomes of such learning, a phenomenon that has been studied as an educational phenomenon for several decades (Goswami; Chen; Dubrawski, 2020; Ward; Pellett; Perez, 2017). It is within this framework that cognitive homeostasis and cybernetics of self-regulation emerge as key issues; the former as a process that capitalizes on the consolidation of learning, and the latter as a pathway to enhance all of the aforementioned through the use of automated digital systems, also referred to as “intelligent” systems.
To conclude the exploration of the previous question, one final key concept emerges: feedback. According to Ackerman, Vance and Ball (2016), self-regulation cannot be effectively accomplished without appropriate feedback. From this perspective, feedback plays a crucial role by providing students with clear guidance on their performance and by giving them specific information about their strengths and areas for improvement, enabling them to make the necessary changes to restore cognitive equilibrium (Lodge et al., 2018).
Within the framework of cybernetics of self-regulation, feedback would be considered a process mediated by intelligent systems, linking teachers, classmates, and students, and providing them with an external and impartial perspective on their performance. This perspective would offer a better-informed condition to correct errors, strengthen knowledge, acquire new skills, reflect on learning, assess progress, and establish realistic goals for the future to achieve cognitive homeostasis.
1.4 Fuzzy logic: addressing the challenges of competency assessment in Assessment 4.0
Considering the above, one of the main challenges lies in developing these intelligent systems that underpin self-regulation and homeostasis processes and enable the generation of effective feedback to support teachers’ work and students’ learning processes.
It is at this point that we introduce both “fuzzy logic” and “competency assessment” as the conceptual framework for the development of these AI-based support systems.
In the mid-1960s, mathematician, and engineer Lotfi Zadeh, considered the father of Fuzzy Logic, proposed its main tenets. According to Jamaaluddin et al. (2019), fuzzy logic is a type of logic that enables reasoning and decision-making in situations involving imprecision or uncertainty. Unlike traditional logic, which uses binary values (true/false), fuzzy logic allows for the representation and management of imprecision and vagueness found in many real-world problems.
Furthermore, Renkas and Niewiadomski (2014) indicate that in fuzzy logic, truth values are expressed in terms of degrees of membership in fuzzy sets, where elements can have partial membership, ranging from 0 to 1, indicating the extent to which an element belongs to the set. This type of partial membership allows for a more suitable representation of uncertainty and imprecision compared to classical logic (Eshuis; Firat; Kaymak, 2021).
Fuzzy logic has been applied in various areas, including artificial intelligence, control systems, decision-making, robotics, and many others (Sousa; Nunes; Lopes, 2015). Its flexibility and ability to deal with uncertainty make it particularly useful in situations where data is incomplete, ambiguous, or subjective, which is often the case in educational processes and, more specifically, in learning assessments.
In this regard, Boychenko et al. (2021) acknowledge the relevance of fuzzy logic for competency assessment processes, as competencies are manifested through performances and are evaluated on a scale, rather than in a binary manner. This means that competence is not simply present or absent but rather developed and positioned at a certain level on the scale at the time of assessment.
Another relevant aspect of fuzzy logic to add to this analysis relates to the fact that competencies are typically assessed using rubrics, which are instruments that consider multiple levels of competence and establish descriptors for each level, allowing for the estimation of the correspondence between descriptors and the performance to be evaluated for a student (Chanchí; Sierra; Campo, 2021). However, as performances are inherently complex, it is common for them to not fully align with a single descriptor or to contain elements from multiple descriptors. This is where fuzzy logic would play a key role in evaluating these performances by considering multiple variables that determine different degrees of membership to various descriptors within the rubric (Rao; Mangalwede; Deshmukh, 2018).
Furthermore, within the current framework of the close relationship between assessment and promotion, where learning assessment ultimately results in a binary pass/fail judgment, allowing students to progress to the next level in their educational journey, it is important to highlight that managing uncertainty and imprecision through the application of fuzzy logic in rubric-based assessment would provide a logical basis for reaching the final binary judgment while accounting for the inherent complexity of student performance.
According to Schembari and Jochen (2013), traditional assessment methods rely on weighted averages and classical logic to measure learning outcomes, legitimizing the learning process in curricula. However, as discussed in this article, fuzzy logic offers a more suitable framework for AI-based assessment systems, given the ambiguous and complex nature of evaluation. Traditional methods often lack sufficient evidence of acquired learning, and when multiple assessors are involved, differences in experience and expertise can lead to varying assessments of the same learning. Fuzzy logic addresses these complexities by offering more nuanced, adaptable decision-making processes.
As an example, Figure 2 presents a case in which a student has a final weighted score of 2.9, which, under classical logic, corresponds to the “Fail” category since, according to institutional rules, a course is passed only if the minimum weighted score is equal to or higher than 3.0. However, in some cases, academic recording systems are programmed to “round up” grades when they are close to passing thresholds, and such processes are even performed directly by instructors in other instances.
Now, what would be the pedagogical argument or reason that a teacher or an academic recording system would have to decide to increase the final grade from two point nine (2.9) to three point zero (3.0) and thereby change the classification from “Fail” to “Pass”? Furthermore, if the previous example included multiple students, assuming that each student has different abilities and limitations inherent to their individuality as individuals, how can we be sure that the reasons for raising or not raising a grade correspond to the realities of these students?
Considering the above, we can indicate that the assessment of a student using classical metrics (weighted averages) particularly reveals two difficulties: firstly, the numerical assessment assigned by an expert using classical metrics can be imprecise and vary among different assessors depending on their experience and socio-affective factors; and secondly, the qualitative assessment using linguistic labels (Pass-Fail; Low, Basic, High, Excellent, etc.) assumes low levels of precision depending on each assessor’s perception and understanding of these labels.
To appropriately address situations like the ones mentioned above, we propose the implementation of an expert recommendation system based on the use of artificial intelligence, through the application of fuzzy logic. This system would enable an “intelligent” and unbiased evaluation of academic and metacognitive processes in students, allowing for an evolutionary, comprehensive, and homeostatic assessment.
In this regard, Figure 3, based on the work of Pitalúa-Díaz et al. (2009), illustrates the stages of reasoning and information processing in a fuzzy expert system. This system consists of four components: a fuzzification interface, a knowledge base, a decision-making unit, and a defuzzification interface.
In the initial stage, the fuzzification interface measures the input variable values of the system and maps them to a fuzzy discourse universe, transferring the range of values to linguistic terms. Fuzzification transforms the input data into linguistic values (Thaker; Nagori, 2018). The second component is the knowledge base, which contains general information about the system. This knowledge base consists of a structured fuzzy database composed of membership functions or degrees of membership to fuzzy sets, assigned intermediate values between zero (0) and one (1), and “if-then” propositions (Chanchí; Sierra; Campo, 2021). Additionally, it includes a set of linguistic rules that control the system’s variables. The third component is the decision-making unit, which simulates the reasoning and logic used by humans when assessing a learning process, (Eshuis; Firat; Kaymak, 2021). Finally, the fourth component is the defuzzification interface, which performs the mapping that transforms the range of output variable values back to their corresponding discourse universes. It converts fuzzy results into understandable numerical values for current academic recording systems (Obregón; Romero, 2013).
In this context, Figure 4 illustrates the variables considered in the design of a fuzzy expert system for assessing learning outcomes based on homeostatic processes inherent in self-regulation cybernetics, through reflective evaluation grounded in evidence. The system consists of two input variables: performance in the final exam (EF-H)(x) and the assessment of the level of metacognition achieved through feedback from a portfolio of evidence provided by students (PM-P)(x). The output variable is the final approval concept (CF)(x).
To represent the degrees of membership of each input variable in the fuzzy sets defined by membership functions, Figure 5 displays the different types of fuzzy sets commonly used to link, match, interconnect, or correspond to the considered fuzzy values. These sets are as follows: Right-shoulder or right-saturation membership function, Left-shoulder or left-saturation membership function, Triangular membership function, Trapezoidal membership function, Gaussian membership function, and Gamma membership function.
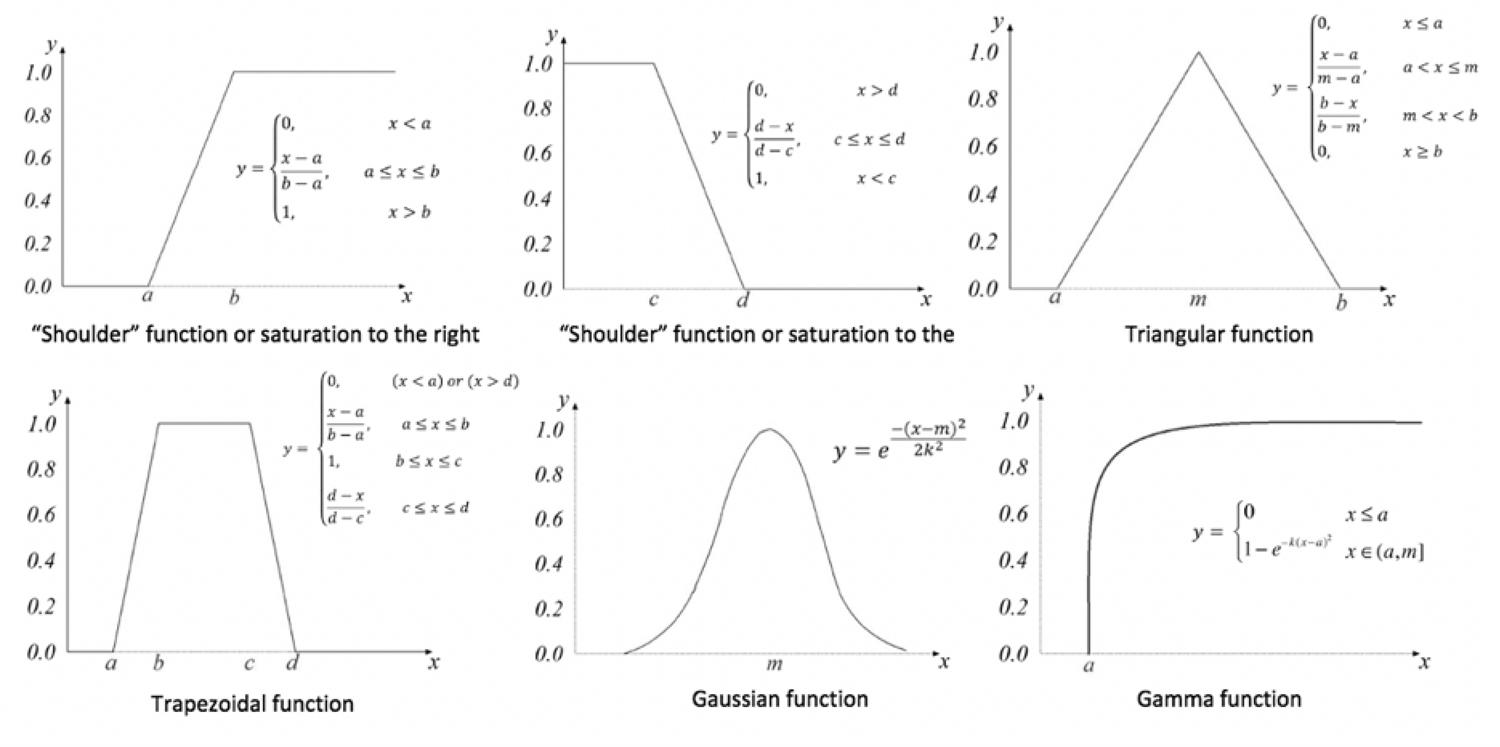
Source: Own elaboration (2024)
Figure 5 Sets of membership or membership functions based on mathematical models.
Figure 6 displays the membership functions of the input and output fuzzy sets of the fuzzy expert system. In this system, right-shoulder and left-saturation, triangular, and trapezoidal membership functions were used.
On the other hand, Table 1 presents a decision matrix for the input and output variables of the system, consolidating the base of sixteen (16) fuzzy rules (expert knowledge). For example, rule number five (5) in the structured fuzzy rule base corresponds to R3: if there is evidence of a “BAD” level in “FINAL TEST” and a “BEST” level in metacognition, then the student is classified as “APPROVED.”
Table 1 Decision matrix for the construction of fuzzy rules of the system
Final Test | |||||
---|---|---|---|---|---|
Metacognition | BAD | AVERAGE | GOOD | BEST | |
BAD | FAILED | APPROVED | APPROVED | APPROVED | |
AVERAGE | FAILED | APPROVED | APPROVED | APPROVED | |
GOOD | APPROVED | APPROVED | APPROVED | APPROVED | |
BEST | APPROVED | APPROVED | APPROVED | APPROVED |
Source: Own elaboration (2024)
On the other hand, Figure 7 illustrates the action of the defuzzification interface, which transforms the output of the fuzzy system into a numerical result for the aforementioned case of a student who has a weighted score of 2.9 on the final exam and is classified as FAILED in the final concept. In the context of a homeostatic and reflective evaluation system based on self-regulation cybernetics that values metacognitive processes, this student is assessed with an additional dimension (metacognition) using a different logic than the classical one (fuzzy logic), resulting in an APPROVED outcome.
In this regard, for the aforementioned case, according to Table 1, which displays the rule base of the fuzzy inference system, rules 1, 2, 3, 4, 5, 6, 7, 8, 11, and 15 have been activated. However, rules three and seven were primarily activated: R3 = if there is evidence of a “BAD” level in the “final exam” (with a definitive grade of 2.9) and a “BEST” level in metacognition (80%), then the student is APPROVED; and rule seven, R7 = if there is evidence of a “BAD” level in the “final exam” (with a definitive grade of 2.9), although it is a low performance according to classical logic, it corresponds much more to a “BASIC” level according to fuzzy logic (see Figure 2), and a “BEST2 level in metacognition (80%), then the student is APPROVED.
1.5 A fuzzy logic-based perspective about Evaluation 4.0
Considering what has been mentioned so far, Evaluation 4.0 can be regarded as a holistic, integral, nonlinear, systemic, evidence-based, and non-standardized process characterized primarily by:
Assessing the degree of interconnection of key knowledge necessary to perform adequately in the era of the Fourth Industrial Revolution, including prior knowledge and 21st-century skills, with the new information perceived by the student when faced with a specific learning situation (Ruíz Martín, 2020).
The implementation of Artificial Intelligence (AI) through the integration of homeostatic intelligent systems, by employing non-classical logic for the assessment and decision-making regarding learning outcomes.
In this regard, this dual circumstance serves as the framework for optimizing the evaluation of learning processes in the context of Education 4.0. Importantly, this gains value when it becomes evident that students, through their executive functions, transition from external feedback to self-feedback, from external regulation to self-regulation, and from external motivation to self-motivation in their learning processes (Bernier; Carlson; Whipple, 2010).
The cybernetics of self-regulation involves training students to explicitly internalize and externalize metacognitive processes by continuously monitoring their learning strategies. This enables them to self-evaluate and reflect on both the strengths and areas for improvement in their study methods. The primary goal of Evaluation 4.0 is to establish an effective self-regulation system, guiding students toward cognitive homeostasis. To achieve this, learners must first identify their learning objectives and motivations. They then plan, organize, and structure their strategies and tools in a systematic manner. Finally, they establish evaluation criteria to independently address challenges throughout the learning process (Martín Celis; Cárdenas, 2014).
2 Conclusions
Given this panorama of inferences and conceptual reflections, it is worth noting the following practical implications of implementing Evaluation 4.0 in the classroom:
Evaluation 4.0 shifts focus from traditional memorization to assessing critical and reflective thinking, where students ask meaningful questions and solve problems creatively. It emphasizes collaborative skills, communication (oral, written, and listening), and essential qualities like perseverance, resilience, self-discipline, and empathy. The goal is to assess what students can do with their knowledge, valuing practical application.
This new approach requires a transformation in traditional evaluation, leveraging artificial intelligence tools like fuzzy expert systems to enhance decision-making in assessing learning outcomes. To implement Evaluation 4.0 effectively, classrooms must first foster a culture of metacognition, encouraging self-assessment and peer evaluation, and allowing time for students to develop metacognitive reasoning. This approach aims to deepen students’ understanding and improve learning strategies.
Ultimately, Evaluation 4.0, from a homeostatic perspective based on the cybernetics of self-regulation, structures and strengthens (in a bio-inspired manner) the mental tools necessary to develop the executive capacities of any citizen in the 21st century, turning the evaluation process into a true learning experience rather than merely an event to validate or verify what has been learned (Noor, 2019). Thus, it configures evaluation as another opportunity for learning.
In the context of Education 4.0, as mentioned by Ramírez-Montoya et al. (2021), the assessment of learning must take a transformative role, aligning with the shifting focus, tools, and methodologies of teaching in the digital age. Moreover, the educational community recognizes that evaluation is not a standalone activity but an integral part of the learning process. In this direction, by embracing a homeostatic approach informed by cybernetics and self-regulation, Evaluation 4.0 acknowledges the interconnectedness of various aspects of learning and leverages this understanding to optimize educational outcomes.
Regarding the above, Taheri, Gonzalez Bocanegra and Taheri (2022) indicate that one significant advantage of Evaluation 4.0 lies in the integration of artificial intelligence, and, from our perspective, if these intelligent systems act like fuzzy expert systems, they will provide educators with enhanced decision-making capabilities, allowing for a more nuanced assessment of metacognitive and cognitive processes. In this sense, by leveraging AI technologies, teachers can gather and analyze comprehensive data, gaining valuable insights into students’ learning progress and tailoring instructional approaches to meet their individual needs (Ovinova; Shraiber, 2019).
Moreover, Evaluation 4.0 extends beyond the traditional concept of assessment as a one-time event with predetermined criteria and recognizes that learning is a dynamic and iterative process, characterized by continuous growth and improvement. Therefore, as mentioned by Oliveira and Souza (2021), assessment of learning in the context of Education 4.0 encourages ongoing self-reflection, self-evaluation, and self-adjustment. Students are actively involved in their learning journey, engaging in metacognitive practices and developing the ability to monitor, regulate, and adapt their learning strategies to achieve optimal outcomes.
Thus, from a prospective research outlook, it is crucial to invest in the development and implementation of intelligent systems that support learning assessment across various educational levels. This involves designing and refining AI-powered tools that can provide accurate and reliable feedback to both students and educators, being versatile enough to cater to diverse student populations, and should align with the specific goals and objectives of different educational contexts.
To ensure the effectiveness of such intelligent systems, rigorous research is required. Studies should explore the impact of AI-powered assessment tools on student learning outcomes, engagement, and motivation and additionally, it is essential to revise the ethical implications of AI integration in Education, ensuring fairness, transparency, and data privacy (Tiwari et al., 2022).
Evaluation 4.0, supported by fuzzy intelligent systems, creates a learning environment focused on continuous improvement and personalized experiences. It equips students with 21st-century skills, promoting active participation, critical thinking, and creative problem-solving. This evaluation method fosters cognitive homeostasis, where students adapt and refine their strategies for optimized learning.
Successful implementation requires a multifaceted approach that emphasizes collaboration, advanced cognitive skills, and the integration of intelligent technologies. Fuzzy expert systems provide personalized feedback, identifying areas for improvement and offering tailored recommendations. However, rigorous research is necessary to evaluate the impact of these technologies and address ethical concerns.
Grounded in homeostasis and self-regulation, Evaluation 4.0 redefines assessment, transforming it into a meaningful learning experience. As we navigate the Fourth Industrial Revolution, investing in intelligent systems and fostering metacognitive practices will prepare learners for future challenges and opportunities.