Serviços Personalizados
Journal
Artigo
Compartilhar
Educação em Revista
versão impressa ISSN 0102-4698versão On-line ISSN 1982-6621
Educ. rev. vol.39 Belo Horizonte 2023 Epub 01-Jul-2023
https://doi.org/10.1590/0102-469837576
ARTICLE
STUDENT DROPOUT IN A BRAZILIAN PUBLIC UNIVERSITY: A SURVIVAL ANALYSIS
1Universidade Federal do Rio de Janeiro. Rio de Janeiro (RJ), Brasil.
The aim of this paper is to analyze whether educational factors and degree choice, sociodemographic origin and academic integration are associated with dropout in the first three years of a student at the Federal University of Rio de Janeiro (UFRJ). We operationalize dropout as the student's permanent departure from the undergraduate course h/she was enrolled. Dropout was analyzed using statistical models of discrete-time survival, which allows treating the data in its longitudinal structure. The institution's microdata, of a census nature, were used for the three-year follow-up of the cohort that entered in the first half of 2014Results point that during the time analyzed, the moments of greatest risk of dropout were the first, second and fifth semester. The sociodemographic origin of the students was not associated with course dropout. Educational and course choice factors were associated with dropout, especially in the first year. The hypothesis is that the course choice process, through the current access format to UFRJ, is possibly structuring an early dropout in the courses. The grade coefficient accumulated per semester (CRa), which was used as a proxy for formal academic integration, was shown to be associated with course dropout. Students with low CRa had a higher risk of dropping out than those with high CRa. These findings reveal the importance of understanding decision-making processes for entering and continuing studies.
Keywords: Higher education; dropout; survival analysis; UFRJ
O objetivo deste artigo é analisar se fatores educacionais e de escolha de curso, origem sociodemográfica e integração acadêmica formal estão associados à evasão nos três primeiros anos do estudante na Universidade Federal do Rio de Janeiro (UFRJ). Empregou-se o conceito de evasão de curso, que é a saída definitiva do estudante do curso de origem. A evasão foi analisada por meio de modelos estatísticos de sobrevivência em tempo discreto, que permitiu tratar os dados em sua estrutura longitudinal. Foram utilizados os microdados da instituição, de caráter censitário, para o acompanhamento de três anos da coorte que ingressou no primeiro semestre de 2014. Os resultados apontam que, durante o tempo analisado, os momentos de maior risco de evasão foram o primeiro, o segundo e o quinto semestre das trajetórias dos estudantes nos cursos. A origem sociodemográfica do estudante não se mostrou associada à evasão de curso. Os fatores educacionais e de escolha de curso se mostraram associados à evasão, principalmente no primeiro ano. A hipótese é de que o processo de escolha de curso, através do atual formato de acesso à UFRJ, possivelmente, esteja estruturando uma evasão precoce nos cursos. O Coeficiente de Rendimento acumulado (CRa), que foi utilizada como proxy de integração acadêmica formal, se mostrou associada à evasão de curso . Os estudantes com CRa baixo apresentaram um risco maior de evadir do que aqueles com CRa alto. Esses achados revelam a importância de entender processos decisórios de entrada e continuação dos estudos.
Palavras-chave: Educação superior; evasão de curso; análise de sobrevivência; UFRJ
El objetivo de este trabajo es analizar si los factores educativos y la elección de cursos, el origen sociodemográfico y la integración académica están asociados con la deserción en los primeros tres años de un estudiante de la Universidad Federal de Río de Janeiro (UFRJ). Se utilizó el concepto de deserción de cursos, que es la salida definitiva del alumno del curso original. La deserción se analizó mediante modelos estadísticos de supervivencia en tiempo discreto, lo que permite tratar los datos en su estructura longitudinal. Los microdatos de la institución, de carácter censal, fueron utilizados para el seguimiento de tres años de la cohorte que ingresó en el primer semestre de 2014. Los resultados indican que, durante el tiempo analizado, los momentos de mayor riesgo de deserción fueron el primer, segundo y quinto semestre de la trayectoria de los estudiantes en los cursos. El origen sociodemográfico de los estudiantes no se asoció con la deserción del curso. Los factores educativos y de elección de cursos se asociaron con la deserción, especialmente en el primer año. La hipótesis es que el proceso de elección de cursos, a través del actual formato de acceso a la UFRJ, posiblemente esté estructurando una deserción temprana en los cursos. El coeficiente de ingresos acumulados (CRa), que se utilizó como proxy de la integración académica formal, se mostró asociada con la deserción de los cursos. Los estudiantes con CRa bajo tenían un mayor riesgo de abandono que aquellos con CRa alto. Estos hallazgos revelan la importancia de comprender los procesos de toma de decisiones para ingresar y continuar estudios.
Palabras clave: Educación superior; deserción; análisis de supervivência; UFRJ
INTRODUCTION
A more demographically and socioeconomically diverse public has started to enter Brazilian higher education in recent decades. The sociological literature has been attentive to the expansion process (PRATES; COLLARES, 2014), the policies implemented to expand access (HERINGER, 2014; SENKEVICS; MELLO, 2019), and the patterns of inequality of opportunities in the sector (RIBEIRO, 2011; MONT'ALVAO, 2011). However, entering higher education is only the first step; the biggest challenge lies in students' persistence and course completion.
Some studies already point to the existence of high dropout rates in Brazilian higher education, and indicate that more recent generations have been facing more difficulties to graduate (RISTOFF, 2013; SILVA FILHO et al., 2007; LOBO, 2012). Thus, in addition to, analyzing student dropout and persistence is important both from a public policy standpoint and for scientific knowledge of educational systems.
This article presents a case study of a university and shows how the educational processes taking place in the university environment follow dynamics that deserve greater attention and emphasis. We intend to contribute academically by presenting ways to think about, measure, and evaluate student dropout in the context of Brazilian higher education. Institutionally this is desirable both at the level of the public higher education system and for the specific institution studied, because we systematize processes that ultimately reflect on the management of the institution and the more efficient use of resources.
In the international literature three sets of factors are pointed out as relevant to understanding dropout and completion in higher education. The first concerns educational dimensions such as students' performance before entering higher education, and variables related to the institution choice process (HILL, 2008; BELASCO, 2013; HOXBY; AVERY, 2013; MEROLLA, 2017). The second set, highlights factors associated with individuals' characteristics - gender, color/race, class, income, parents' education - that would be associated with individuals' path and outcomes before and after entering higher education (BUCHMANN et al., 2008; BOWEN; CHINGOS; MCPHERSON, 2009; GOLDRICK-RAB, 2006). Finally, the third set of factors, highlights the importance of students' experiences while attending higher education, especially formal academic integration - usually measured by students' performance during their studies - and social engagement (TINTO,1975, 2012; BRAXTON et al., 2004; CHARLES et al., 2009).
These factors can be analytically distinguished, but from an empirical point of view, they are treated as complementary. Based on this literature, this paper examines whether factors - educational and course choice, students' social and demographic background, and formal academic integration - are associated with dropout in the first three years at the Federal University of Rio de Janeiro (UFRJ). We employed the concept of course dropout, which is the student's definitive departure from the course of origin (LOBO, 2012).
We treat the student dropout process as a temporal problem, that is, the factors that lead to dropout accumulate over time or only appear at a certain point in the trajectory (TINTO, 1975, 2012). In the language of research designs this means that methodologically studies ideally should be longitudinal rather than cross-sectional. The difficulty of implementing longitudinal research designs is that they demand detailed tracking of student cohorts entering higher education systems and institutions. If conducting population surveys is no longer a trivial task at one point in time, doing it for the same set of people repeatedly adds new layers of complexity. Traditional surveys have a central concern of maintaining the representativeness of the sample relative to the population (HENRY, 1990). This unfolds into concerns in the construction of the sample (coverage rates, response rates, selection of observation units) and of the questionnaire (quality of application, reliability and validity of the questions asked) (BARNETT, 1991; GROVES, 2009). In longitudinal surveys, the need to obtain responses from the same individuals over time causes the problems of sample representativeness to be raised again at each new round of research, which continues to have he challenge of not having biases with the additional challenge of not losing respondents (SINGER; WILLET, 2003).
In this research we overcome this type of problem by using administrative records which can be defined as data that derive from the operation of information systems typically originating from public agencies (ELIAS, 2014). Typically, these data are collected for the purpose of recording transactions in the provision of services (WOOLLARD, 2014). The administrative records of the UFRJ enrollment system are the source of information for our research. Since the information is generated from the university sector that monitors the academic situation of students, the problem of temporal monitoring of respondents, typical of longitudinal research is circumvented, since the students' own daily administrative acts (enrolling, graduation, enrollment suspension) are our source of information. Additionally, the representativeness of our data is guaranteed because we use census information rather than a sample, that is, we treat the universe of students enrolled at the university who entered in the first semester of 2014.
In addition to the information from the administrative records of the UFRJ enrollment system, we also used variables from the socioeconomic questionnaire that is produced and applied by the institution during student pre-enrollment. This instrument presents high response rates, especially regarding variables of social and demographic origin of the students, and a diversity of questions such as socioeconomic, cultural, school, and choice aspects and expectations about the course that are not found in public and national databases.
This data structure allows us to explore the temporal dimensions of the dropout process. Crucially, we identify when students are most at risk of dropping out of courses. To answer time-related questions, longitudinal models of survival analysis are considered more appropriate (SINGER; WILLET, 2003), and applied more frequently to study dropout in international research. In general, these studies seek not only to know "when" higher education dropout or completion occurs, but also, seek to identify which are the factors associated with these outcomes and when they act (AMERI et al., 2016; LASSIBILLE; GÓMEZ, 2008; GURY, 2011).
In Brazil, studies working with longitudinal modeling including time in their equations to study dropout in higher education are still scarce. Therefore, this research contributes to studies on higher education in Brazil by adopting a sparsely mobilized design and a data analysis suitable to incorporate the structure of the data (see methods section). We thus produce evidence about a cohort of students that entered UFRJ in the first semester of 2014 , and the patterns of association of a set of individual factors of student origin, educational and course choice aspects, and formal academic integration for the study of course dropout.
This paper is divided into seven sections including this introduction. The second section contextualizes Brazilian higher education and also the Federal University of Rio de Janeiro (UFRJ). The third, brings a brief review of international and national literature. The fourth section of the article describes the data, and the fifth section describes the method used in the research. The sixth section brings the results and discussions, and in the seventh and last section the final considerations.
BRAZILIAN HIGHER EDUCATION AND THE FEDERAL UNIVERSITY OF RIO DE JANEIRO
In recent decades, Brazilian higher education has undergone an expansion under several aspects, such as: growth in the number of institutions, increase in vacancies and enrollment, among others (SANTOS et al., 2020). During the years from 2003 to 2015, the expansion of this level of education was related to the democratization of the Brazilian campus. In this period, a set of policies and programs were implemented, aiming at the inclusion of sectors historically excluded from higher education (RISTOFF, 2014).
Initiatives such as: the ProUni and the Fies, both aimed at expanding access to private higher education; the Enem/Sisu; the Reuni; the adoption of affirmative action policies; the implementation of Law No. 12.711/12 in federal institutions of higher education (Ifes) are typically cited as relevant in this context (SENKEVICS, 2021; ALMEIDA, 2015). Among these measures, we will focus on the Enem/Sisu, because this initiative has modified the access to the Ifes and changed the dynamics of the course choice process, an important factor to think about the dropout of courses and understand the trajectories of students after enrolling, as already pointed out by some studies (MEROLLA, 2017; ELLER; DIPRETE, 2018; NOGUEIRA et al., 2017).
The Enem was created in 1998, during the government of Fernando Henrique Cardoso, to evaluate the performance of students graduating or leaving high school. In addition, its results began to serve as a form of access to higher education. In 2009, the Ministry of Education (MEC) proposed to the Ifes that they use Enem as a test for selecting candidates instead of their own selection processes (formely known as vestibulares). For this, the MEC, through the National Institute of Educational Studies and Research Anísio Teixeira (INEP), conducted a methodological restructuring of the exam so that it could fulfill its new purpose (LUZ; VELOSO, 2014).
Along with these reformulations in Enem, it was established, in 2010, the Unified Selection System - Sisu. Instituted and regulated by Normative Ordinance No. 2 of January 26, 2010, the Sisu is a computerized system managed by the MEC for the selection of candidates for vacancies in undergraduate courses offered by participating public institutions of higher education.
This system is structured in successive stages, which are constituted in the following phases: I- offer of vacancies in Sisu by the participating institutions, available for the enrollment of candidates; II- enrollment in Sisu of candidates who have participated in Enem from the previous year's edition; III- selection and classification of candidates based on their performance on Enem, observing the weights and minimum scores eventually established by the institution; IV- release of vacancies occupied in Sisu (BRASIL, 2010).
Sisu can be described as a matching mechanism, i.e., a type of auction that allow for adjustments between the vacancies offered by the institutions and the individuals who compete for them (ABREU; CARVALHO, 2014). During the registration period, the student, in a five-day interval, can, at any time, access the Sisu platform and choose, in order of preference, up to two course options among those offered by the institutions participating in the process. From the second day on, the system uses each student's options, "computing the matching" that would be offered to them in the next stage. After a few hours, the student, when accessing the system platform, receives information about the "computed matching" (ABREU; CARVALHO, 2014).
These simulations allow students to know if they would be selected in the first call to enroll in their first or second course option, or if they would not be selected. In this process, they can observe, for each of the course options, their Enem score, the score of the last classified, and also their position in the ranking (ABREU; CARVALHO, 2014). These adjustments happen four times: if the registration period began on a Monday, the student will receive signals of the choices of other competitors daily from Tuesday until Friday, the last day of registration (ABREU; CARVALHO, 2014).
As soon as the student announces the vacancy he or she will apply for, on the last day, the system computes a matching based on the last list of courses announced by the student and, based on this adjustment, makes vacancy offers. If the candidate has a score to be classified in his two course options, he will be selected only for the first, because each candidate competes only for his first course option, passing to compete for the second only if he does not get a place in the first (BRASIL, 2010). In addition, when classified and receiving a vacancy offer, the student can choose to accept or reject it.
It is important to point to the change in the dynamics of course choice in the selection process caused by Sisu, to the extent that, in the traditional entrance exam (vestibular), the individual applied for a course and then took the test in which he was only approved if he achieved sufficient performance to pass (NOGUEIRA, et al. 2017). In Sisu, the individual first takes Enem to obtain a score and then applies to two courses, as a first and second option (NOGUEIRA, et al. 2017).
We understand that it is fundamental to consider the form of access to higher education to think about the of students' dropout from undergraduate courses. As the international literature points out (MEROLLA, 2017; ELLER; DIPRETE, 2018), pre-entry factors in higher education are key to understanding students' trajectories once they are enrolled. What was their previous performance? How is the institution chosen, by convenience, low cost, expectation of high financial returns (AINA, 2011; HOXBY; AVERY, 2013)? In the following sections we will operationalize some of these dimensions and scrutinize them empirically to understand their importance in the UFRJ dropout process.
As federal universities have autonomy to choose the format of their selective processes, it is important to take into consideration highlight the context of changes in access to the Federal University of Rio de Janeiro. Until 2008, UFRJ still selected its students through the traditional entrance exam (vestibular). This exam consisted of two-day discursive tests. The novelties in the entrance exam for undergraduate courses began to appear in the selection process that took place in 2009 for admission of the 2010 class. That year, the selection began to be carried out in two stages: in the first stage, exclusively eliminatory, UFRJ adopted Enem; in the second stage, UFRJ continued to adopt its own entrance exam (vestibular).
In 2011, UFRJ established policies to reserve spaces for students from public schools, based on a selection process that combined the results of Enem with the institution's entrance exam (vestibular). In 2012, UFRJ determined the end of the vestibular and the exclusive access to undergraduate courses through Enem/Sisu. In addition, that year, the institution increased to 30% the percentage of vacancies reserved for students from public schools and the inclusion of family income as a second criterion for disputing these vacancies. However, with the approval of the Quotas Law No. 12.711, on August 29, 2012, all federal higher education institutions should immediately implement in 2013 the Law, which provided a quota of at least 25% of vacancies for students that completed their secondary education in public high schools. Within this quota, other criteria such as income and racial self-identification were implemented. The law also imposed, a deadline of four years for full compliance with this law, i.e. 50% of vacancies per course and shift, reserved for students who completed high school in public schools (BRASIL, 2012).
As of 2014, the UFRJ began to reserve 50% of vacancies in each course for affirmative action modalities and the other 50% for broad competition. Thus, we highlight that the cohort of students analyzed in this article entered only through Enem/Sisu and approximately half of the students of this cohort entered through some category of quota, due to the sanction of Law No. 12.711/12.
LITERATURE REVIEW
Factors associated with dropout in higher education: what the international literature tells us.
There are several factors associated with dropout in higher education. We highlight three lines with strong presence in the literature: educational factors and choice of higher education institution; factors associated with students' sociodemographic backgrounds; students' experiences within Higher Education Institutions (HEI) settings.
The first line of analysis proposes that investigating students' educational trajectories and the choice process prior to entering higher education helps in understanding future educational outcomes, such as dropout and completion. According to Eller and DiPrete (2018) the distribution of entrants' characteristics is not homogeneous across a number of factors structuring the educational experience in higher education. For example, students' prior educational achievement is highly associated with higher education completion (MEROLLA, 2017). Research highlights that minority students experience comparatively less academic preparation for higher education due to quality in elementary education and lack of guidance, from school, community, and family members, during the college application process (BELASCO, 2013; HILL, 2008). Underprivileged students' inexperience with the selection processes, and unmet expectations regarding the feasibility of college options, are factors associated with higher dropout rates (DEIL-AMEN; DELUCA, 2010; JENNINGS et al., 2015; PERNA, 2006).
Moreover, the universities' selection process can influence choices, since students tend to self-select. In other words, the student chooses to enroll in institutions that require scores on entrance exams compatible with his or her performance (MANSKI; WISE, 1983). Translating to the Brazilian and UFRJ case, students, depending on their Enem scores, apply or not to certain institutions and courses through Sisu. Their choices may influence their future outcomes, of either dropping out or completing the degree, since they do not represent the first choice of course or the desired institution. This self-selection mechanism may indicate that the student's behavior favors the transition to higher education regardless of the course, since this is chosen based on what is possible according to academic performance, which does not always coincide with what is desired. As far as we could identify, this is an explanatory path not much used in the context of Brazilian research on higher education dropout and completion. Later on, we will explore it as a possible source to address the empirically verified patterns of dropouts at UFRJ.
The second line of study examines how markers of students' social and demographic background, such as gender, color/race, class, or family socioeconomic status are associated with the outcomes of either dropping out or completing higher education.
As for gender, a perceived trend is the presence of a gap in higher education completion that favors women when compared to men (BUCHMANN; DIPRETE; MCDANIEL, 2008). Currently, in many countries, including Brazil, higher proportions of women than men complete tertiary education (BUCHMANN; DIPRETE; MCDANIEL, 2008; MENDES et al., 2020). Among the 30 member countries of the Organization for Economic Cooperation and Development (OECD), the predominant male advantage in college completion has disappeared in all but four countries - Switzerland, Turkey, Japan, and Korea (BUCHMANN; DIPRETE; MCDANIEL, 2008). Although women generally outnumber men in their numerical representation and also in their persistence in higher education, they are still concentrated in less prestigious institutions or in less profitable fields of study (GERBER; CHEUNG, 2008).
Another important factor of student background, and one that may influence inequalities in educational outcomes, is color/race. International research indicates that bachelor's degree completion is racially stratified, in that whites complete more often than black students (BOWEN; CHINGOS; MCPHERSON, 2009; ROKSA et al., 2007). In this regard, Snyder et al. (2016) indicate that in the U.S., 63% of white students complete a bachelor's degree within six years of initial college entry, while the completion percentage for blacks is 41%. Eller and DiPrete (2018) indicate that the discrepancy in academic and socioeconomic resources at college entry between whites and blacks is an important associated factor in explaining the racial gap in U.S. higher education completion rates. Once they enter college, white students have an easier time than black students integrating socially and academically into institutions. This is because academic and socioeconomic resource advantages are racially structured in favor of whites, making it an important explanatory factor in understanding racial inequality in higher education completion (ELLER; DIPRETE, 2018).
Evidence also points out that family socioeconomic status (SES), as measured by family income and/or parental education, is associated with student dropout outcomes in higher education (GOLDRICK-RAB, 2006; ZARIFA et al., 2018). In the case of public universities in the United States, research shows that the relationship between parental education and graduation rates remains even after controlling for other student characteristics (BOWEN; CHINGOS; MCPHERSON, 2009). Students from families with low socioeconomic status have consistently higher odds of dropping out than their peers from privileged families (BOWEN; CHINGOS; MCPHERSON, 2009). In European universities, undergraduate students with parents with educational attainment lower than higher education have higher odds of dropping out (AINA, 2013; CONTINI et al., 2018). In the UK parental occupation is statistically significant in explaining completion and dropout (JOHNES; MCNABB, 2004). Overall, studies provide evidence that the category at highest risk of dropping out is students with parents in underprivileged socioeconomic positions, i.e., having parents with lower education and lower income increases the dropout risk rate (AHLBURG et al., 2002).
Finally, studies focused on students' experiences point out that integration processes between individual and institution are factors that are associated with dropout and completion of higher education. In the field of Sociology, there are some theoretical models that seek to explain dropout in higher education. Most sociologically oriented models share the view expressed by Spady (1970, 1971), who was one of the first theoretical models in this literature that propose that social integration plays a key role in interacting with institutional satisfaction and commitment and thus affects the decision to drop out. Among the models that share Spady's view, the most extensively examined and empirically tested are those of Tinto (1975, 1993) and Pascarella (1980), both developed in the United States (AINA, 2013).
Tinto (1975, 1993) refines and modifies the model posited by Spady (1970, 1971) and argues that the process of dropout can be viewed as a temporally structured process of interactions between the individual and the academic and social system of the university, during which the student's experiences continually change leading to adaptations of their institutional goals and commitments in ways that lead to persistence or varied forms of dropout. Tinto's model emphasizes process-based interactions between individual student attributes and institutional structures within the university, and the mechanism that would structure permanence would be integration into the school community (TINTO, 1975; 2012).
Pascarella (1980) also focuses on student integration with the university environment. For this author, students' interactions with the institution play a key role in shaping academic experiences. However, not all interactions are equally valuable: informal interactions with faculty contribute more to committing students to the institution in which they are enrolled, leading them to complete their degree. The quality of such interactions depends on the one hand, on individual and family characteristics (personality, ability, aspirations, family, and home environment, etc.) and, on the other hand, on the characteristics of the faculty and the institution (size, culture, organization, etc.). The intersection between students and institutional characteristics shapes students' decisions and achievements.
Charles et al. (2009) focus on the different experiences among white, black, Hispanic, and Asian students. In this paper the authors argue that two parallel processes can explain student persistence: a predominantly social process whereby students gain satisfaction and become attached to the institution, and a primarily academic process of achievement whereby students earn good grades and continually accumulate credits toward graduation. The authors conclude that social engagement is more predictive than academic achievement in decisions not to drop out of higher education.
Braxton et al. (2004) point out that academic achievements are important for persistence in college. Unlike Charles et al. (2009), these authors argue that social engagement is not as central to student persistence. This is due to the multiple competing priorities that typically shape the lives of students attending institutions other than large research universities. Therefore, the authors suggest that the quality and type of institution interact with students' backgrounds to determine which university-level experiences-academic or social-are most important to undergraduate completion outcomes.
National literature review: dropout in Brazilian higher education.
Dropout in Brazilian higher education is not a new problem, however, until the beginning of the first decade of the 21st century there were not very many productions on this theme in Brazil (BAGGI; LOPES, 2011). In recent years, research on the subject has begun to appear more frequently, probably because of the processes of change that marked Brazilian higher education during the period, such as the expansion of the sector and the implementation of policies and programs to democratize access (MACIEL et al., 2019, SANTOS JUNIOR; REAL, 2017). In addition, it can be observed that there are conceptual and methodological divergences in the national literature and that there are unfilled gaps in the works that cover the theme of dropout and completion of higher education degrees in the country (VITELLI; FRITSCH, 2016). Below, we present some works with the aim of contextualizing our research problem and sustain that the contributions of the present study are significant to the state of knowledge on dropout in national research, especially due to our longitudinal research design, the statistical modeling and the analytical factors presented above.
Most Brazilian studies follow a descriptive, cross-sectional and exploratory approach of the issues regarding dropout and completion, without the mobilization of multivariate statistical techniques. At the national level, it is known that the average annual dropout rate of in-person courses from 2000 to 2005 was approximately 22% (SILVA FILHO et al., 2007). There is an important differential between private (26%) and public (12%) HEIs. Among the Brazilian regions, the North is the one that presented the lowest annual dropout rate from 2000 to 2005 (SILVA FILHO et al., 2007).
Although less recurrent, there are case studies that analyze dropout in private institutions. Evidence shows that students who benefited from the University for All Program (ProUni) are less likely to drop out compared to those who did not benefit (FELICETTI; FOSSATTI, 2014). In addition, failing grades, increased tuition, pending payments, and an increase in relative age increase the chances of dropping out (SILVA, 2013).
In Science, Mathematics, and Computing and Engineering, Production, and Construction, nationally, for a generation of higher education entrants in 2009, course dropout is higher at private institutions than at public ones (SACCARO et al., 2019). Also, being male and older decreases the individual's persistence in higher education, while students who are contemplated with financial support show longer tenure in courses (SACCARO et al., 2019).
A case-study focused on a Business course, in a specific federal university found that student’s performance, the number of semesters in the course, gender, as well as experiencing course failure and enrollment suspension , are factors that explain both the length of stay and the risk of dropout (COSTA et al., 2018). However, the variables related to age at entry, marital status, color/race, and the nature of the school in basic education (public or private) showed no influence on the time to completion or dropout (COSTA et al., 2018).
In the case of the Federal University of Rio de Janeiro, a study for cohorts of entrants in 2012 and 2013 showed that there is no substantive difference in evasion between blacks and whites, i.e., whites evade as much as blacks (COSTA; PICANÇO, 2020). In addition, those who tend to drop out more are men, as well as entrants with a spouse, with children, who worked at the time of entry, who were from the night shift, and who had low socioeconomic indices and low cultural capital (COSTA; PICANÇO, 2020).
The present article differs from these works in that it studies a more recent cohort of entrants to UFRJ, 2014, in a longitudinal manner in order to follow students throughout their undergraduate trajectory. In addition, this research applies a multivariate model that considers time in its equation, a strategy that has been little mobilized by research on dropout in Brazil to verify students' dropout risk (COSTA et al., 2018; SACCARO et al., 2019; SILVA, 2013). Also, we have advanced in analyzing the effects of course choice variables, performance at the entrance to UFRJ, which is the Enem score, in addition to performance throughout the trajectory of students in the course, which is the cumulative Coefficients of Achievement per semester. As far as we could identify, these resources have been little mobilized in the Brazilian literature on the subject, despite being adequate, recommended, and highly used in similar research contexts in debates about dropping out in other countries.
Thus, the main questions this article sets out to investigate given that the international literature on dropout highlights the importance of exploring the temporal dimensions of the dropout process, we want to know: when students are most at risk of dropping out of a course? Given studies that highlight that educational achievements are largely determined by people's social backgrounds, we ask: are students' background factors associated with course dropout at UFRJ? Taking into account the current selection process adopted by the institution (Enem/Sisu) and that course choice can influence future outcomes, we investigate: are educational factors, such as Enem performance, and course choice associated with course dropout and how their effect varies along students' undergraduate trajectory? Research shows that more academically integrated, high-achieving students are less likely to drop out, so we as: is formal academic integration associated with dropping out at UFRJ? Does its effect varies along the undergraduate trajectory?
DATA
The database used in this study was built from the microdata of the cohort of first semester 2014 entrants at UFRJ, provided by the Student Registration Division (DRE-PR1) through a formal request and a term of ethical commitment. Most of the information is collected through a socioeconomic questionnaire produced and applied by the institution at the time of pre-enrollment, therefore, these data can be classified as secondary, since we did not create them. The aforementioned questionnaire is composed of 46 questions that address, among other information, socioeconomic, cultural, school, family composition and choice aspects and expectations about the course and the institution. The students are followed by their enrollment number, de-identified throughout their trajectory within the course. It is not possible to identify specific students due to privacy protocols that were implemented in the data. The student's relationship with the university in each semester can be classified as: enrolled, enrollment suspended, enrollment cancelation, degree conclusion, re-enrollment, exchange student, and student in academic mobility. The population of this study is composed of those entering in the first semester of 2014. A total of 4982 students are in the database. We chose to analyze only courses in the in-person modality and on the Rio de Janeiro city campus. In addition, it was necessary to exclude courses that had a maximum duration of 2 years to avoid bias in the analysis. After removing these observations (496 cases), we arrived at a total of 4486 students followed over three years.
The dependent variable used in this analysis is a binary variable that informs if the student persisted or dropped out of UFRJ. We operationalized the concept of as the student who leaves the course of origin for any reason (LOBO, 2012). This variable was constructed by combining the variable of time that the individual remained in the course and the enrollment status in each semester. We considered only those students who had their enrollment in the course cancelled, except for cancellation due to degree conclusion. We chose to cut off the time until the 6th semester, that is, the first three years of the students' trajectories, to include all the courses, regardless of their duration. This cut-off is justified for empirical reasons, to meet the assumptions of statistical modeling, but it is also justified by the literature, given that international and national studies (SACCARO et al., 2019; SILVA, 2013; AMERI et al., 2016; LASSIBILLE; GÓMEZ, 2008; GURY, 2011) evidence that the risk of dropout in the first years of the student is higher. This means that our data are "censored to the right," meaning that we do not know if a student dropped out of UFRJ after three years. All interpretations must take these assumptions into account.
Independent variables. Several studies highlight that factors associated with individuals' characteristics - gender, color/race, parents' education - are associated with individuals' trajectories and outcomes after entering higher education (BUCHMANN et al., 2008; BOWEN; CHINGOS; MCPHERSON, 2009; GOLDRICK-RAB, 2006; ELLER; DIPRETE, 2018; AINA, 2013; etc.). Therefore, as students' sociodemographic background variables we used color/race (white=0; black and brown=1), gender (female=0; male=1), and family socioeconomic position (PSE), measured by the highest parental education of the students' father or mother (less than higher education=0; higher education=1). Taking into account the current selective process adopted by UFRJ (Enem/Sisu), and that the process of choices in accessing higher education can influence future outcomes (LARSEN et al, 2013; ZWICK, 2009; PERNA, 2006; MEROLLA, 2017), we used as variables representing educational and course choice factors the information "if it was the first course choice" (yes=0; no=1) and "if the cutoff score determined the course choice" (no=0; yes=1), as well as, the Enem score divided into five performance levels separated from the quintiles. Research shows that more academically integrated students, with good performances, are less likely to drop out (TINTO, 2012; BRAXTON et al., 2004; BENNETT, 2009; SUHRE et al. 2007; ARAQUE et al. 2009; BELLOC et al. 2010). Therefore, as a proxy for the student's formal academic integration into the course, we use the cumulative Coefficient of Achievement (CRa) per semester divided into five levels separated from the quintiles. The CRa is the overall performance of a student, measured by the weighted average of the final grades obtained in each subject, using as weight the number of credits (class hours) that the subject confers. Having a good CR implies getting scholarships, internships, opportunities at the institution, which can influence the students' experiences after entering the university. A course variable, covering a combination of 45 UFRJ courses, was added to the model as a control for heterogeneity.
Other independent variables from the socioeconomic questionnaire were tested in the descriptive statistical and multivariate analyses, such as age, type of high school attended, whether they had children, whether they were working at the time of entry, and whether they had to move to another city to study. These variables were not statistically significant in any of our analyses and including them in the model would decrease our population because of missing data.
In Table 1, we present descriptive statistics of the cohort analyzed. As for the general profile of the students, for the individual characteristics’ color/race, gender and family PSE, this cohort is distributed in approximately 50% for each category of these variables. As for the course choice factors, that is, the students who said it was not their first choice of course and that the cutoff score "yes" affected their choice of course, it was approximately 25%.
As for the dropout and persistence rates of students, for the variables family PSE and gender, the statistical test showed that there is a small difference of 4 percentage points between the categories of each variable (male x female, P value = 0.003; higher education x<than higher education, P value = 0.002). For the color/race variable, no difference was observed between the white x black and brown categories (P value = 0.564). For the course choice variables, the statistical test indicated a difference of 18 percentage points between the categories "no x yes"(P value = 0.000). Also, for the variables of Enem score and CRa, we noticed differences between the percentages of the categories (P value = 0.000). Here we have an initial indication that there is greater variation in dropout among the variables we call "educational and course choice" than among the social origin and demographic variables. In the results section we will assess whether this conclusion holds up from a multivariate exercise.
Table 1: Descriptive statistics of the 2014.1 entering cohort at UFRJ.
Full sample | Dropped out until 6th semester | Persisted | Totals | ||||||
---|---|---|---|---|---|---|---|---|---|
N | % | N | % | N | % | N | % | ||
Family SES | Higher Education | 2,237 | 51.51 | 658 | 30.60 | 1,492 | 69.40 | 2,150 | 100% |
< Higher Education | 2,106 | 48.49 | 709 | 35.31 | 1,299 | 64.69 | 2,008 | 100% | |
Color/race | White | 2,241 | 53.38 | 711 | 33.04 | 1,441 | 66.96 | 2,152 | 100% |
Black and Brown | 1,957 | 46.62 | 605 | 32.46 | 1,259 | 67.54 | 1,864 | 100% | |
Sex | Male | 2,161 | 48.16 | 731 | 35.61 | 1,322 | 64.39 | 2,053 | 100% |
Female | 2,326 | 51.84 | 692 | 30.85 | 1,551 | 69.15 | 2,243 | 100% | |
First course choice | Yes | 3,335 | 74.94 | 904 | 28.37 | 2,283 | 71.63 | 3,187 | 100% |
No | 1,115 | 25.06 | 504 | 46.93 | 570 | 53.07 | 1,074 | 100% | |
Cutoff score affected course choice | Yes | 1,133 | 25.46 | 514 | 47.24 | 574 | 52.76 | 1,088 | 100% |
No | 3,317 | 74.54 | 894 | 28.18 | 2,279 | 71.82 | 3,173 | 100% | |
ENEM score - fifths of performance | 1 (Low) | 884 | 19.71 | 268 | 30.32 | 616 | 69.68 | 884 | 100% |
2 | 941 | 20.98 | 328 | 34.86 | 613 | 65.14 | 941 | 100% | |
3 | 955 | 21.29 | 357 | 37.38 | 598 | 62.62 | 955 | 100% | |
4 | 906 | 20.20 | 293 | 32.34 | 613 | 67.66 | 906 | 100% | |
5 (High) | 800 | 17.83 | 177 | 22.13 | 623 | 77.88 | 800 | 100% | |
Cumulative Performance Coefficient per semester - fifths of performance | 1 (Low) | 1,383 | 30.97 | 885 | 63.99 | 498 | 36.01 | 1,383 | 100% |
2 | 749 | 16.77 | 176 | 23.50 | 573 | 76.50 | 749 | 100% | |
3 | 841 | 18.84 | 153 | 18.19 | 688 | 81.81 | 841 | 100% | |
4 | 687 | 15.39 | 90 | 13.10 | 597 | 86.90 | 687 | 100% | |
5 (High) | 805 | 18.03 | 116 | 14.41 | 689 | 85.59 | 805 | 100% |
Source: UFRJ / DRE/PR1. Elaborated by the author.
METHOD
To incorporate time into the study and take advantage of the longitudinal design of the data we used a discrete-time survival analysis method. This modeling strategy is used to estimate the time until a given event occurs (SINGER; WILLETT, 2003). These models are suitable for investigating whether the event occurs, when it occurs, and the factors associated with the occurrence or non-occurrence of the event and its temporal heterogeneity.
Singer and Willett (2003) point out three methodological features that need to be defined to conduct a survival analysis: the target event whose occurrence is being studied, the beginning of time, which is an initial starting point when no one in the study has experienced the target event, and the metric for time, which is a meaningful scale at which the occurrence of the event is recorded. In this research, the target event is course dropout, the event occurrence is the transition from course enrollment status to "cancelled," the starting point of time is entry into the university - 2014.1 cohort, and the metric for time of the event occurrence is recorded by semester. Thus, in the language of these models, time is treated as a discrete metric.
Survival methods differ from traditional statistical techniques in that they provide a way to simultaneously analyze the times of event occurrence and non-occurrence of the event. This is done through the hazard function and the survival function. The occurrence of the event in each discrete period is known as the hazard. Denoted by h (tᵢⱼ), the discrete-time hazard is the probability that individual i will experience the event in period j, given that he did not experience it in any previous period. Conditionality ensures that the hazard represents the probability of the event occurring among individuals eligible to experience the event in that period. As individuals experience the event, they drop out of the hazard set and are not eligible to experience it in later periods. Due to this conditionality, the hazard probability for individual i in period j assesses his unique hazard of event occurrence in that period (SINGER; WILLETT, 2003). The hazard function assists in identifying especially risky time periods, that is, when the event is most likely to occur. In addition, it shows whether the hazard increases, decreases, or remains constant over time.
Allied to the hazard function, the survival function provides another way to describe the distribution of event occurrence over time. Unlike the hazard function, which assesses the single hazard associated with each period, the survival function accumulates these occurrence risks, period by period, to assess the probability that a randomly selected individual will "survive," that is, not experience the event. The survival function is defined as the probability that individual i survives period j. For this to happen, the individual must not experience the target event in the jth period or any previous period (SINGER; WILLETT, 2003). It is important to point out that at the beginning of the process observation, when no one has yet experienced the event, everyone is surviving and, by definition, its value is 1. Over time, as events occur, the survival function decreases.
To find out the hazard between the occurrence of the event at each time period and the predictors, we use logistic regressions to regress the event indicator (dependent variable) on the time indicators (time dummies) and the selected predictors (independent variables) on the data set in person-period format, also known as the long data format. This means that the observations are nested in the individuals and repeated in the rows by the amount of time the individual remains under observation.
Importantly, the discrete-time hazard base model invokes a common but restrictive assumption: that each predictor has an identical effect across all periods studied. This restriction, known as the proportionality assumption, stipulates that the effect of a predictor does not depend on the respondent's duration in the initial state. However, it is possible that the effect of a particular predictor is not stable over time, so a non-proportional model may be more appropriate. This was the case in this research.
The interactions of time with predictors are justified by bivariate analyses and through fit statistics (SINGER; WILLETT, 2003). Breaking the proportionality assumption, can be done by including statistical interactions by adding a cross-product term to a model that already includes the main component effects so that the factors associated with the event can vary over time. Our bivariate exploratory analyses showed that of the predictors analyzed those that did not follow proportional measures over time were: first course choice, grade affected course choice, Enem and CRa, so for these we added interaction with time. The equationbelow represents our model choice:
The main time effect is represented by D₁ to D յ and the interaction between X₁ and time is represented by the terms X₁ D₁ to Xյ to Dյ.
RESULTS
As mentioned in the method section, the hazard and survival functions allow us to check both sides of the phenomenon, the occurrence of the event that shows the riskiest moments of dropping out of the course, and the non-occurrence of the event that shows the proportion of students who survived. The key tool for summarizing the population distribution of event occurrence is the life table, a table that tracks the event histories of individuals from the beginning of time counting, when no one has yet experienced the target event, to the end of data collection. Table 2 presents a life table for the cohort of students who entered UFRJ in 2014.1.
Table 2: Life table for cohort of students who entered UFRJ in 2014.1
SURVIVAL | ||||||
---|---|---|---|---|---|---|
Time | Interval | Total | Deaths | Survival | Standard Error | [95% Conf. Int.] |
1 | 1 2 | 4486 | 358 | 0.9202 | 0.0040 | 0.9119 0.9278 |
2 | 2 3 | 4128 | 328 | 0.8471 | 0.0054 | 0.8362 0.8573 |
3 | 3 4 | 3800 | 179 | 0.8072 | 0.0059 | 0.7953 0.8184 |
4 | 4 5 | 3621 | 97 | 0.7856 | 0.0061 | 0.7733 0.7973 |
5 | 5 6 | 3524 | 332 | 0.7115 | 0.0068 | 0.6980 0.7246 |
6 | 6 7 | 3192 | 129 | 0.6828 | 0.0069 | 0.6690 0.6962 |
HAZARD | ||||||
Time | Interval | Total | Cumulative. Failure | Hazard | Standard Error | [95% Conf. Int.] |
1 | 1 2 | 4486 | 0.0798 | 0.0798 | 0.0042 | 0.0718 0.0883 |
2 | 2 3 | 4128 | 0.1529 | 0.0795 | 0.0044 | 0.0711 0.0883 |
3 | 3 4 | 3800 | 0.1928 | 0.0471 | 0.0035 | 0.0405 0.0543 |
4 | 4 5 | 3621 | 0.2144 | 0.0268 | 0.0027 | 0.0217 0.0324 |
5 | 5 6 | 3524 | 0.2885 | 0.0942 | 0.0052 | 0.0843 0.1046 |
6 | 6 7 | 3192 | 0.3172 | 0.0404 | 0.0036 | 0.0337 0.0477 |
Source: UFRJ / DRE/PR1. Elaborated by the author.
The results in Table 2 show that the semesters with the highest hazard of dropping out in the period of time analyzed are the first and second, configuring the student's first year at the university, and the fifth semester. As for survival, we notice that at the end of the first year 84% of the students in the analyzed cohort remained in their original courses, and at the end of the 6th semester 68% continued in their courses.
Both international and national studies (SACCARO et al., 2019; SILVA, 2013; AMERI et al., 2016; LASSIBILLE; GÓMEZ, 2008, GURY, 2011) evidence highest hazard rates of dropout in the first year of the course, so we realize that UFRJ follows in the direction of the evidence already found by other researchers. This result points to a kind of "tasting" period of the course and university environment by the students, since approximately 15% of students drop out in the first year, it is relevant to investigate how the conditions of choice of course and the format of entry into the university structure the dropout.
As this is a cohort that entered the university via the Enem/Sisu selection process, this seems to us an important result, since the Sisu induces an adaptive choice of the student based on his or her grade, and not an early choice as it occurred with previous selection processes such as the vestibular. The high dropout rate at the beginning of the course signals that the students' choices can be made with two references: to enter or not the university, and only afterwards in which course to enter, as we will show in the next analyses.
The peak hazard rate of dropout in the fifth semester seemed counterintuitive to us. Through descriptive exploration, we have conjectured the hypothesis that the high hazard of dropping out in the fifth semester may be related to a process that arises administratively (in the data) by the rules of the institution (cancellation of enrollment and other processes), for example the accumulation of locked semesters that results in the cancellation of enrollment.
Multivariate analysis
To find out whether the hazard rate between the occurrence of dropout over time is associated with students' origin, educational and course choice factors, and formal academic integration, we conducted a multivariate analysis applying the discrete-time survival model. The modeling process resulted in 11 models in which we added groups of variables and chose the best model according to Akaike's Information Criterion (AIC), as shown in table 3. We emphasize that the table with the coefficients of the final model can be found in the appendix of this article, as well as the post-estimation tests.
Table 3: AIC Fit Statistics
Model 1 |
Model 2 |
Model 3 |
Model 4 |
Model 5 |
Model 6 |
Model 7 |
Model 8 |
Model 9 |
Model 10 |
Model 11 |
|
---|---|---|---|---|---|---|---|---|---|---|---|
AIC | 9491812 | 9479091 | 9284950 | 9267834 | 8107544 | 7878265 | 7878313 | 7858264 | 7860819 | 7857950 | 7730043 |
Source: UFRJ / DRE/PR1. Elaborated by the author.
Thus, the model chosen and the results presented below are taken from model 11, which contains the dummies of time, family PSE, color/race, gender, interaction of time with the variable "cutoff score affected the choice of course", interaction of time with the first course option, interaction of time with the quintile of the ENEM score, interaction of time with the quintile of the accumulated CR per semester, and the variable of course dummies to control for heterogeneity. To facilitate interpretation, we transformed the coefficients into predicted hazard rates, based on the calculation of adjusted predicted probabilities using Stata's margins command (LONG; FREESE, 2014).
We emphasize that the results of the predicted risk of dropout for the variables color/race, gender, and family PSE, were presented as proportional associations in time. By adding the interactions of the time dummies with the predictors first course choice, grade affected course choice, Enem and CRa, it was possible to verify, and therefore read, the results of the predicted hazard of dropout for these variables in each semester.
Are students' social and demographic background factors associated with course dropout at UFRJ?
Through the variables of color/race, gender and family PSE, we can check whether there are indications of inequalities of results according to the social and demographic origin of the students. Chart 1 shows the predicted hazard rates of dropping out of the course according to color/race, controlling for the other independent variables. We observe that white students had a slightly higher hazard rates of dropping out of the course than black and brown students. However, the small difference between the groups' predicted hazard rates is not statistically significant at any point in time, as verified in the post-estimation tests (test: t1= -0.006; t2 =-0.008; t3= -0.005; t4= -0.003; t5= -0.008; t6= -0.005; P value>0.05). This result indicates that whites drop out as much as blacks and browns and goes in the same direction as other Brazilian studies that found no direct relationship of color/race in the dropout of students from courses (COSTA et al., 2018; COSTA; PICANÇO, 2020).
As for gender, controlling for the other variables, women presented a slightly higher hazard rate risk of dropping out of the course than men, as shown in graph 2. However, the small difference between the groups' predicted values is not statistically significant at any point in time, as verified in the post-estimation tests (test: t1= -0.006; t2 = -0.008; t3= -0.005; t4= -0.003; t5= -0.008; t6= -0.005; P value>0.05). Thus, for this UFRJ cohort the findings indicate that women drop out as much as men.
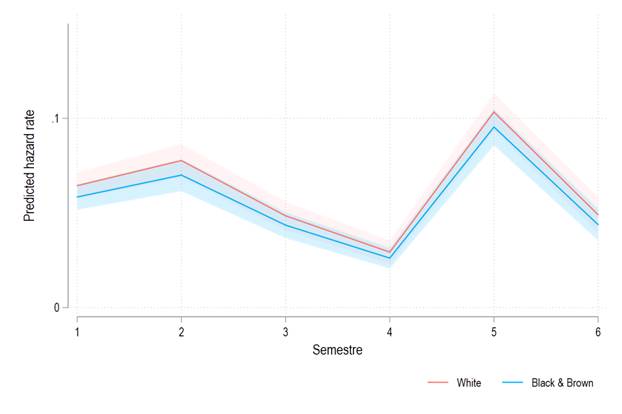
Source: UFRJ / DRE/PR1. Elaborated by the author.
Chart 1: Predicted hazard rates of dropping out of a course according to color/race.
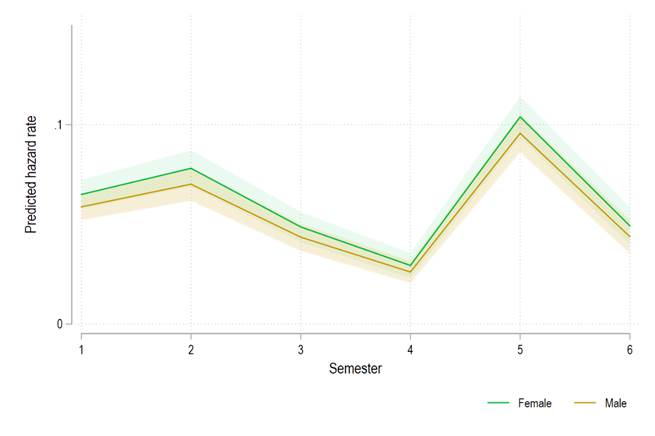
Source: UFRJ / DRE/PR1. Elaborated by the author.
Graph 2: Predicted hazard rate of course dropout according to gender.
The socioeconomic position of the family measured by the maximum education of the parents is presented in graph 3. Controlling for the other variables, students with parents with less than higher education (<ES) had a hazard rate of dropping out very close to students with parents with higher education (>=ES). The difference between the groups' predicted values is not statistically significant at any point in time, as verified in the post-estimation tests (test: t1= -0.005; t2 = -0.007; t3= -0.004; t4= -0.003; t5= -0.007; t6= -0.005; P value>0.05). That is, for this cohort at UFRJ, there is no direct association of family socio-economic position and course dropout.
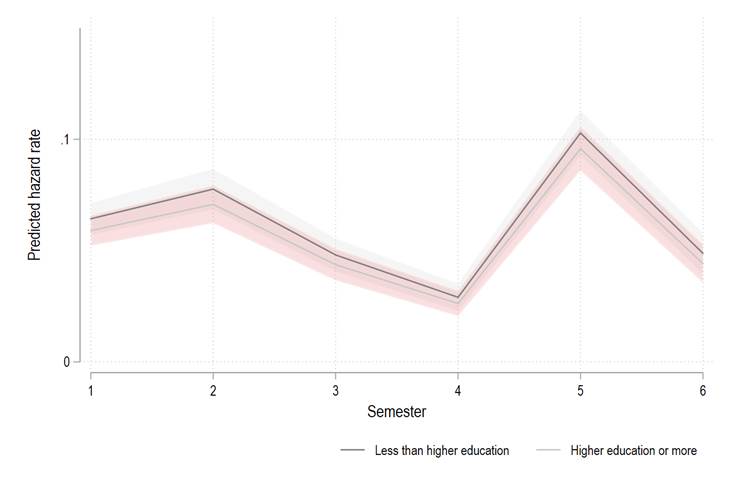
Source: UFRJ / DRE/PR1. Elaborated by the author.
Chart 3: Predicted hazard rate of dropping out of the course according to the family's socioeconomic status.
These results indicate little difference between the categories of the students' socio-demographic variables. They should be interpreted considering both the extensive controls we used, as well as the socioeconomic selectivity at the entrance of a university that adopts a competitive selection process. Since UFRJ is a selective university, one hypothesis is that social selection took place at the entrance of the institution, that is, at the transition from high school to higher education, or even in the previous educational stages.
This type of result is known in the sociology of educational stratification which indicates that social backgrounds influence each of the educational transitions differently (MARE, 1980, 1981). In this sense, Shavit and Blossfeld (1993) point out that educational systems open up from the bottom up, so that cohorts successively and slowly advance within the educational hierarchy. However, the highest educational levels remain fairly exclusive, with the result that real bottlenecks are created in the transition to the top level (SHAVIT; BLOSSFELD, 1993). This process is meaningful both from a statistical and sociological perspectives since it creates more homogeneous populations according to various sociodemographic and educational dimensions. We also caution that we insert students' courses in the models as dummy variables. This is equivalent to treating courses as fixed effects in our modeling strategy. Fixed effects are typically included to control for unobserved heterogeneity across different levels of the fixed effects variable and they essentially capture the average effect of these unobserved factors on the dependent variable. Once we take this heterogeneity in consideration in conjunction with other controls, we find that the socio-demographic factors have little differences. How course specific characteristics shape dropout rates and how they act in the suppression or amplification of individual characteristics is a topic that deserves attention in another paper.
Are educational and course choice factors associated with dropping out at UFRJ? Does this result vary along the student's trajectory in undergraduate studies?
Before entering university there is an educational and choice process that can influence future outcomes (PERNA, 2006, HOXBY; AVERY, 2013; MEROLLA, 2017). Thus, we analyzed the association of course dropout with the variables "cutoff score affected course choice," "first course choice," and Enem performance quintile, controlling for the other factors.
Chart 4 shows that students who said the cutoff score affected their choice of course had a higher risk predicted hazard rate of dropping out compared to those who said the cutoff score did not affect their choice. We observed that the difference between the groups was greater at the end of the student's first year in the course, that is, in the second semester (t2). As verified in the post-estimation test, the difference between groups was statistically significant in all semesters except the 6th (test: t1= 0.049; t2 = 0.079; t3= 0.024; t4 = 0.034; t5= 0.062; P value<0.01; t6= 0.012; P value>0.05).
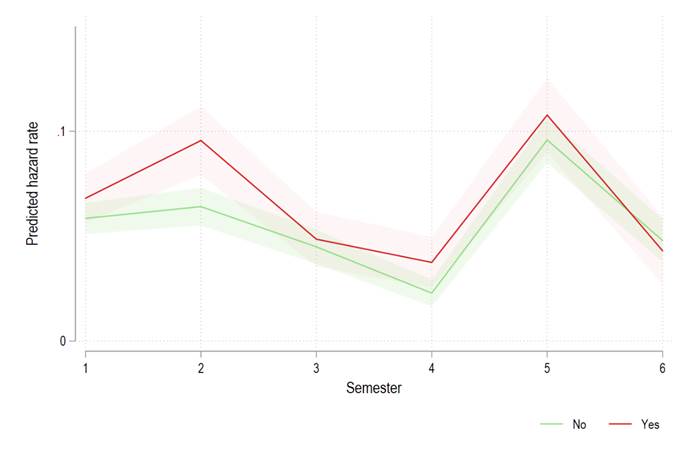
Source: UFRJ / DRE/PR1. Elaborated by the author.
Graph 4: Predicted hazard rate of course dropout according to whether score affected course choice over time.
As for the variable first course option, we noticed in Graph 5 that the predicted hazard ratios of dropping out were higher for those who said the course was not their first choice, compared to those who said the course was their first choice, controlling for the other factors. According to the post-estimation test, the difference between the groups was statistically significant in all semesters except the 3rd and 6th (test: t1= 0.066; t2 = 0.092; t4 = 0.030; t5= 0.050; P value<0.01; t3= 0.013; t6= 0.014; P value>0.05). We observed that the association was higher in the first and second semester.
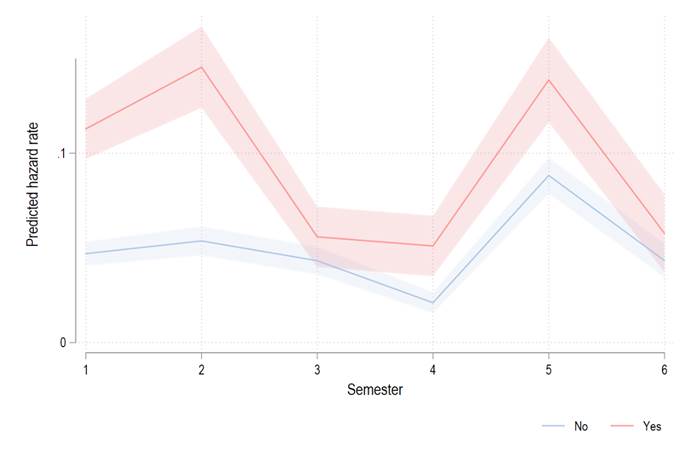
Source: UFRJ / DRE/PR1. Elaborated by the author.
Graph 5: Predicted hazard rate of course dropout according to first course choice over time.
Thus, these course choice variables give us indications about the selection process used by UFRJ in the analyzed period, that is, the Enem/Sisu. Some studies have already indicated that Sisu modifies the dynamics of course choice (NOGUEIRA et al., 2017). In Sisu, the student first take the Enem exams and only after knowing the score applies for a first and second course option. In this sense, Nogueira et al. (2017) state that through simulations made in the initial stage of Sisu the student tests their chances of being approved in certain courses and institutions. Therefore, the system, even if indirectly, stimulates the adjustment of original preferences to what is possible even if it is not the desired course or institution (NOGUEIRA et al., 2017). Thus, the high risk of dropping out in the first year of the course, of those that the course option was not the first and that the cutoff score affected the choice, signals that possibly getting into university was more important for this cohort of students than where to enter.
As for Enem performance, graph 6 shows that the students who had the highest risk of dropping out, in the first year of the course, were those with Enem performance in the 2nd, 3rd and 4th quintiles, compared to those with low performance, i.e., in the 1st quintile, and high performance, in the 5th quintile. In the first year only the differences between the predicted hazard rates from the 2nd to the 1st quintile and from the 5th to the 4th quintile were statistically significant (P value <0.05).
Interestingly, in the students' first year in the courses, those who had lower Enem performances, i.e., in the 1st quintile, had a low dropout risk, close to that of those with high performance, in the 5th quintile. One hypothesis for this result, according to the literature, is that students with lower scores have little opportunity for mobility/choosing another discipline of study, leading them to place a higher value on their place at university (MAY; BOUSTED, 2004).
We noticed that the predicted hazard rate of the performance categories changed across semesters and that after the first year, those with performance in the 5th quintile had lower hazard of dropping out of courses.
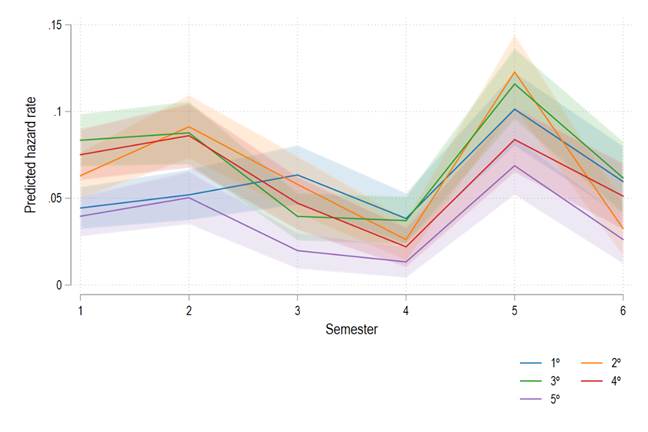
Source: UFRJ / DRE/PR1. Elaborated by the author.
Chart 6: Predicted hazard rate of dropping out according to the quintile of performance in ENEM over time.
Therefore, we observed that the association of educational and course choice factors with dropping out are greater than the relation of students' social and demographic origins. The results of the multivariate analysis indicated that, controlling for the other variables, educational and course choice factors would be associated with course dropout in the analyzed cohort, especially in the student's first year. Again, we refer to the Sisu and its induction of the course choice logic. We emphasize that the change in the selection process was not accompanied by curricular changes in the courses, which continue with the same structure prior to the implementation of the new selection process (Enem/Sisu). It is important to point out that students make their choices in an iterative and adaptive way. When they enter university, most of the time they find courses structured in careers and subjects in a rigid way, which can contribute to dropout in the beginning of the course.
Is formal academic integration associated with course dropping out at UFRJ? Does this result vary along the student's undergraduate trajectory?
To analyze dropout in a higher education institution, we know that it is important to distinguish students' experiences within the academic and social systems of institutions (TINTO, 2012). The academic system is composed of characteristics directly linked to the educational experiences of students, such as their performance in the courses, attendance, and other experiences etc. The social system, on the other hand, has informal characteristics, such as personal relationships and involvement among members of the institution, especially the contacts between students in meeting places within the university. Thus, the academic integration of students is directly linked with the formal characteristics of the system and the social integration with the informal characteristics (TINTO, 2012).
The socially integrated student is not necessarily academically integrated and vice versa. For example, a student can be integrated into the social system of the university, have good relationships with colleagues and employees of the institution, participate in specific groups organized by their peers, and yet drop out because they are not academically integrated, i.e., they cannot maintain attendance, good grades, etc. (TINTO, 2012). However, it is important to point out that the impact of academic integration and social integration is not necessarily symmetrical, it depends very much on each higher education institution (BRAXTON et al., 2004). Maintaining an adequate level of performance in the academic system, in most higher education institutions, is the minimum formal condition for student permanence.
Thus, in this study we prioritized the measurement of formal academic integration since it was not possible to obtain information about the social integration of students. Thus, this was measured by the student's accumulated coefficient of income per semester (CRa).
Chart 7 shows that students with low CRa (1st quintile) were at higher hazard of dropping out in the entire period analyzed, compared to students in the other performance quintiles. Verification by the post-estimation test indicates that the difference between the predicted hazard from the 1st quintile to the other quintiles is statistically significant (P value <0.05).
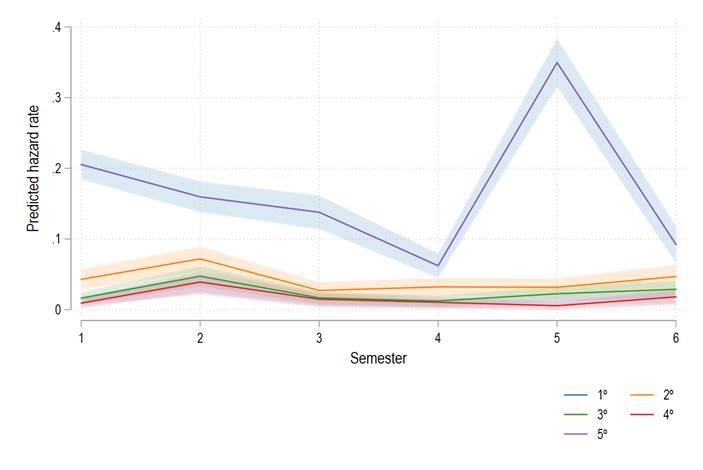
Source: UFRJ / DRE/PR1. Elaborated by the author.
Chart 7: Predicted hazard rate of course dropout according to CRa quintile over time.
This result shows that in the cohort and during the time analyzed, the students who had accumulated coefficient of income per semester (CRa) in the upper quintiles were those who presented lower predicted hazard rates of dropping out of the course. Having a good CRa implies getting scholarships, internships, opportunities at the institution, which may influence students' experiences after entering the university.
Several studies take students' academic achievement as a proxy for formal academic integration and point out that the higher the student's grades, during university studies, the lower the risk of dropping out (BENNETT, 2009; SUHRE et al., 2007; ARAQUE et al., 2009; BELLOC et al., 2010).
Also, research highlights that students' first academic achievements significantly influence the likelihood of dropping out, in other words, students are more likely to continue at university if they get good grades early on (BELLOC et al., 2010; BENNETT, 2009; MONTMARQUETTE et al., 2001; PASCARELLA; TERENZINI, 2005).
However, one must be cautious about whether CRa is in fact associated with difficulties in following the course or not, or whether it is mediated by other factors that were not observed: learning, motivation, social integration, etc. In addition, there is the limitation that the peak hazard rate in the fifth semester may be capturing a process that arises administratively (in the data) by the rules of the institution. In practice the student may have dropped out before the institution registering the departure.
FINAL CONSIDERATIONS
This paper showed that in students' first three years, the hazard rates of dropping out of the course is highest at the beginning of the courses and then in the fifth semester. Multivariate analysis revealed some counterintuitive results. White students drop out as much as black and brown students when we incorporate a multivariate analysis that incorporates individual level variables as well as the course that students are enrolled. The difference between women's predicted hazard rates of dropping out was not statistically significant compared to men. Students with parents with less than a college education had a hazard rate of dropping out of the course close to students with parents with a college education. Therefore, in aggregate for this cohort of UFRJ students, students' social and demographic background was not shown to be associated with course dropout. These results need to be interpreted taking into consideration that entering an academically selective higher education institution selects students that are more motivated and academically prepared
Nonetheless, we were able to identify educational factors and the choice of course were associated with dropping out, especially in the first year. The predicted hazard rates of those who reported that the cutoff score affected their choice of course was higher than the hazard of those who said the cutoff score did not affect their choice. Also, the hazard rates of students who said the course was not their first choice was higher than those who said the course was their first choice. As for the Enem performance quintile, the students who presented the lowest hazard of dropping out in the first year of the course were those with performance in the 1st quintile and in the 5th quintile. This result changed throughout the semesters analyzed and showed that students with high performance (5th quintile) were more resilient than the others. Another variable that was shown to be associated with course dropout was the Cumulative Coefficient of Achievement (CRa) which was used as a proxy for formal academic integration. Students with low CRa had a higher hazard of dropping out than those with CRa in the top quintiles.
We emphasize that the results verified in this article stem from a case study of a cohort of UFRJ students and cannot be extrapolated to other cohorts and institutions. Thus, we caution that all results should be interpreted as typical of the institution. In the language of case studies (GERRING, 2007), we cannot state whether the studied context is typical, diverse, extreme, deviant, influential. We argue that this issue - known as a problem about the external validity of the study - is counterbalanced by the high quality of the data, that is, internal validity, the availability of a research design that allows for the incorporation of time (longitudinal data, rarely available in the Brazilian research context) and availability of the measurement of variables that point to important mechanisms in structuring the interruption and continuity of studies in a large research university.
Also, we point out that the modeling technique opted for an aggregate analysis of all students across courses. Thus, for future studies, it is necessary to ascertain how these factors affect dropout in a disaggregated and course specific way, since there are different undergraduate courses, and the heterogeneity that permeates the institution was not known.
Even with these limitations, our analysis allows us to make some considerations regarding the cohort analyzed. We found an early dropout period, in the first year of the course, which may be working, on the part of the student, as a period to experience how the course and the university works and decide to stay or drop out of the course. As educational and course choice factors showed greater influences in the student's first year, our hypothesis is that the process of course choice, through the current format of access to the university, is possibly structuring an early dropout in the courses. Even if indirectly, Enem/Sisu induces an adaptive choice of the student based on his/her score, and not an early choice as it occurred in the vestibular. These results indicate that efficiency gains in the system as a whole, which may have occurred with the adoption of Sisu - cost reduction for students, centralization of the selection - are counterbalanced by efficiency loss at the institution level.
Based on the results, some indications can be made. First, most energy should be put into establishing precautions/measures to reduce dropout in the early stages of courses, because that is where the issues of student dropout and transfer are most pertinent.
Another issue to be considered, to try to improve the formal academic integration of the student, is to carry out curricular adaptation in the first semesters of the courses. Some international research shows that several higher education institutions try to avoid problems - such as too many demands at the beginning of the course, too many exams, too much material for study - by offering better conditions for students, reforming curricula, offering transition courses to compensate for educational gaps, and making mentors and tutors available for the first year (LARSON et al., 2013; HEUBLEIN, 2014).
Anyway, these are just a few suggestions among many that can be studied according to the case of each higher education institution to decrease course dropout and increase student graduation. Along with the creation and implementation of measures to reduce dropout, it is essential to consider the monitoring and evaluation of the impact of these initiatives.
REFERENCES
ABREU, Luís; CARVALHO, José. Análise do jogo induzido pelo mecanismo SISU de alocação de estudantes em universidades. In: ENCONTRO NACIONAL DE ECONOMIA, 42., 2014, Natal, RN. Anais.Natal, RN: ANPEC, 2014. Disponível em:Disponível em:https://www.anpec.org.br/encontro/2014/submissao/files_I/i863b19702151c4ea9c41924b9a7a7b9d8.pdf . Acesso em:10/05/2020. [ Links ]
AHLBURG, Dennis; MCCALL, Brian; NA, In-gang. Time to dropout from college: A hazard model with endogenous waiting. Working Paper N°. 0102, Industrial Relations Center, University of Minnesota, 2002. Disponível em: Disponível em: http://www.legacy-irc.csom.umn.edu/RePEC/hrr/papers/0102.pdf Acesso em:05/07/2021. [ Links ]
AINA, Carmen. Parental background and university dropout in Italy. High Educ 65, pag.437-456, 2013. <https://doi.org/10.1007/s10734-012-9554-z> [ Links ]
ALMEIDA, de Wilson Mesquita. “Os Herdeiros E Os Bolsistas Do Prouni Na Cidade De são Paulo”. Educação & Sociedade 36, no. 130 (2015): 85-100. [ Links ]
AMERI, Sattar; CHINNAM, Ratna; FARD, Mahtab; REDDY, Chandan. Survival Analysis based Framework for Early Prediction of Student Dropouts. INTERNATIONAL CONFERENCE ON INFORMATION AND KNOWLEDGE MANAGEMENT, 16, Indianapolis, IN, USA 2016 <DOI: 10.1145/2983323.2983351> [ Links ]
ARAQUE, Francisco; RÓLDAN, Concepción; SALGUERO, Alberto. Factors influencing university drop out rates. Computers & Education, n. 53, p. 563-574, 2009. [ Links ]
BAGGI, Cristiane; LOPES, Doraci. Evasão e avaliação institucional no ensino superior: uma discussão bibliográfica. Avaliação, Campinas, v. 16, n. 2, p. 355-374, 2011. <https://doi.org/10.1590/S1414-40772011000200007> [ Links ]
BARNETT, Vic. Sample Survey Principles and Methods. London: Edward Arnold, 1991. [ Links ]
BELASCO, Andrew. Creating College Opportunity: School Counselors and Their Influence on Postsecondary Enrollment. Research in Higher Education 54(7), p. 781-804, 2013. http://dx.doi.org/10.1007/s11162-013-9297-4 [ Links ]
BELLOC, Filippo; MARUOTTI, Antonello; PETRELLA, Lea. University drop-out: an Italian experience. Higher Education, v. 60, n. 2, p. 127-138, 2010. [ Links ]
BENNETT, Roger. Determinants of Undergraduate Student Drop Out Rates in a University Business Studies Department. Journal of Further and Higher Education, v. 27, n. 2, p.123-141, 2009. [ Links ]
BRASIL. Lei n.º 12.711, de 29 de agosto de 2012. Dispõe sobre o ingresso nas universidades federais e nas instituições federais de ensino técnico de nível médio e dá outras providências. Diário Oficial da União, Brasília, DF, 30 ago. 2012. Seção 1, p. 1-2. [ Links ]
BRASIL. Portaria Normativa n.º 2, de 26 de janeiro de 2010. Institui e regulamenta o Sistema de Seleção Unificada, sistema informatizado gerenciado pelo Ministério da Educação, para seleção de candidatos a vagas em cursos de graduação disponibilizadas pelas instituições públicas de educação superior dele participantes. Diário Oficial da União, Brasília, DF, jan. 2010. Seção 1. [ Links ]
BRAXTON, John; HIRSCHY, Amy ; MCCLENDON, Shederick. Understanding and Reducing College Student Departure. ASHE-ERIC Higher Education Report. San Francisco, CA: Wiley Periodicals, 2004. [ Links ]
BOWEN, William, CHINGOS, Matthew; MCPHERSON, Michael. Crossing the Finish Line: Completing College at America’s Public Universities. Princeton, NJ: Princeton University Press, 2009. [ Links ]
BUCHMAN, Claudia; DIPRETE, Thomas; MCDANIEL, Anne. Gender Inequalities in Education. Annual Review of Sociology 34, p. 319-37, 2008.<https://doi.org/10.1146/annurev.soc.34.040507.134719> [ Links ]
CHARLES, Camille; FISCHER, Mary; MOONEY, Margarita; MASSEY, Douglas. Taming the River: Negotiating the Academic, Financial, and Social Currents in Selective Colleges and Universities. Princeton, NJ: Princeton University Press, 2009. [ Links ]
CONTINI, Dalit; CUGNATA, Federica; SCAGNI, Andrea. Social selection in higher education. Enrolment, dropout and timely degree attainment in Italy. High Educ 75, p.785-808, 2018. <https://doi.org/10.1007/s10734-017-0170-9> [ Links ]
COSTA, Andréa; PICANÇO, Felícia. Para além do acesso e da inclusão: impactos da raça sobre a evasão e a conclusão no Ensino Superior. Novos estudos CEBRAP [online], v. 39, n. 2, pp. 281-306, 2020. <https://doi.org/10.25091/s01013300202000020003> [ Links ]
COSTA, Francisco; BISPO, Marcelo; PEREIRA, Rita . Dropout and retention of undergraduate students in management: a study at a Brazilian Federal University. RAUSP Management Journal [online], v. 53, n. 1pp. 74-85, 2018. <https://doi.org/10.1016/j.rauspm.2017.12.007> [ Links ]
DEIL-AMEN, Regina; DELUCA, Stefanie. The Underserved Third: How Our Educational Structures Populate an Educational Underclass. Journal of Education for Students Placed at Risk (JESPAR) 15(1-2), pp.27-50, 2010.<https://doi.org/10.1080/10824661003634948> [ Links ]
ELIAS, Peter. Administrative Data. In: DUSA, A.; NELLE, D.; STOCK, G.; WAGNER, G. (Orgs.). Facing the Future: European Research Infrastructures for the Humanities and Social Sciences. Berlin: SCIVERO, 2014, p.47-48. [ Links ]
ELLER, Chistina; DIPRETE, Thomas. The Paradox of Persistence: Explaining the Black-White Gap in Bachelor’s Degree Completion. American Sociological Review. Article first published online. Volume: 83 issue: 6, pp. 1171-1214, 2018.<https://doi.org/10.1177/0003122418808005> [ Links ]
FELICETTI, Vera Lucia; FOSSATTI, Paulo. ProUni and current students in licentiate undergraduate courses: dropouts in focus. Educar em Revista [online]. n. 51, pp. 265-282, 2014. <https://doi.org/10.1590/S0104-40602014000100016> [ Links ]
GERBER, Theodore; CHEUNG, Sin Yi. Horizontal stratification in postsecondary education: forms, explanations, and implications. Annual Review of Sociology, v. 34, pp. 299-318, 2008.<https://doi.org/10.1146/annurev.soc.34.040507.134604> [ Links ]
GERRING, John. Case Study Research : Principles and Practices. New York: Cambridge University Press, 2007. [ Links ]
GOLDRICK-RAB, Sara. Following Their Every Move: An Investigation of Social-Class Differences in College Pathways. Sociology of Education 79(1), pp. 61-79, 2006. Disponível em:<Disponível em:http://www.jstor.org/stable/25054302 > Acesso em:01/07/ 2021. [ Links ]
GROVES, Robert M. Survey Methodology. 2nd ed.Wiley Series in Survey Methodology. Hoboken, N.J: Wiley, 2009. [ Links ]
GURY, Nicolas. Dropping out of higher education in France: a micro‐economic approach using survival analysis. Education Economics, 19, pp.51-64, 2011.<DOI:10.1080/09645290902796357> [ Links ]
HENRY, Gary T.. Practical Sampling. Newbury Park, CA: Sage, 1990. [ Links ]
HERINGER, Rosana. Um balanço de 10 anos de políticas de ação afirmativa no Brasil. Tomo: Revista do Programa de Pós-Graduação e Pesquisa em Sociologia Sergipe, n. 24, p. 17-35, 2014.<https://doi.org/10.21669/tomo.v0i0.3184> [ Links ]
HEUBLEIN, Ulrich. Student drop-out from German higher education institutions. European Journal of Education, v. 49, n. 4, p. 497-513, 2014. [ Links ]
HILL, Diane. School Strategies and the ‘College- Linking’ Process: Reconsidering the Effects of High Schools on College Enrollment. Sociology of Education, 81(1), p.53-76, 2008.<https://doi.org/10.1177/003804070808100103> [ Links ]
HOXBY, Caroline M.; Christopher AVERY. The Missing ‘One-Offs’: The Hidden Supply of High - Achieving, Low-Income Students. Papers on economic activity, Brookings Institution, Washington, DC, pp.57-66, 2013. Disponível em:<Disponível em:https://www.brookings.edu/wp-content/uploads/2016/07/2013a_hoxby.pdf >. Acesso em : 20/08/2021. [ Links ]
JENNINGS, Jennifer; DEMING, David; JENCKS, Christopher; LOPUCH, Maya, SCHUELER, Beth. Do Differences in School Quality Matter More Than We Thought? New Evidence on Educational Opportunity in the Twenty- First Century. Sociology of Education, 88(1), pp.56-82, 2015. <https://doi.org/10.1177/0038040714562006> [ Links ]
JOHNES, G.; MCNABB, R. Never give up on the good times: Student attrition in the UK. Oxford Bulletin of Economics and Statistics, 66, pp.23-47, 2004.<DOI: 10.1111/j.1468-0084.2004.00068.x> [ Links ]
LARSEN, Michael S.; KORNBECK, Kasper Pihl.; KRISTENSEN, Rune; LARSEN, M. R.; SOMMERSEL, Hanna Bjornoy. Dropout phenomena at universities: what is dropout? why does dropout occur? what can be done by the universities to prevent or reduce it? Technical report, Danish Clearinghouse for educational research, 2013. [ Links ]
LASSIBILLE, Gérard; GÓMEZ, Lucía. Why do higher education students drop out? Evidence from Spain. Education Economics, 16:1, pp.89-105, 2008. <DOI:10.1080/09645290701523267> [ Links ]
LOBO, Maria Beatriz. Panorama da evasão no ensino superior brasileiro: aspectos gerais das causas e soluções. Cadernos ABMES, v. 25, p. 9-58, 2012. Disponível em:<Disponível em:https://abmes.org.br/arquivos/publicacoes/Cadernos25.pdf >. Acesso em:01/01/2021. [ Links ]
LUZ, Jackeline; VELOSO, Tereza. Sistema de seleção unificada (SISU): refletindo sobre o processo de seleção. Revista Educação e Fronteiras, Dourados, v. 4, n. 10, p. 68-83, jan./abr. 2014. Disponível em: <Disponível em: https://ojs.ufgd.edu.br/index.php/educacao/article/view/3649 >. Acesso em: 03/05/2021. [ Links ]
MACIEL, Carina Elisabeth; CUNHA, Mauro e Lima SILVA, Tatiane. A produção científica sobre permanência e evasão na educação superior no Brasil. Educação e Pesquisa [online], v. 45. p. e198669, 2019. <https://doi.org/10.1590/S1678-4634201945198669>. [ Links ]
MANSKI, Charles; WISE, David. College Choice in America. Cambridge: Harvard University Press, 1983. [ Links ]
MARE, Robert. Social Background and School Continuation Decisions. Journal of the American Statistical Association, 75:370, p.295-305, 1980. <DOI: 10.1080/01621459.1980.10477466.> [ Links ]
MARE, Robert. Trends in Schooling: Demography, Performance, and Organization. The Annals of the American Academy of Political and Social Science, 453(1), p.96-122, 1981. <doi:10.1177/000271628145300105> [ Links ]
MAY, Steve; BOUSTED, Mary. Investigation of student retention through an analysis of the first-year experience of students at Kingston University. Widening participation and lifelong learning, v. 6, n. 2, p. 42-48, 2004. [ Links ]
MENDES, Tayná; HOUZEL, Luiza; MILANSKI, Bruna; et al. Azul ou rosa? A segregação de gênero das escolhas educacionais no ensino superior brasileiro, 2002-2016. Agosto 26, 2020. Available at SSRN:<https://ssrn.com/abstract=3682320> or <http://dx.doi.org/10.2139/ssrn.3682320> [ Links ]
MEROLLA, David. Completing the Educational Career: High School Graduation, Four-Year College Enrollment, and Bachelor’s Degree Completion among Black, Hispanic, and White Students. Sociology of Race and Ethnicity, 4(2), p.281-97, 2017.<https://doi.org/10.1177/2332649217727552> [ Links ]
MONT’ALVÃO, Arnaldo. Estratificação educacional no Brasil do Século XXI. Dados: Revista de Ciências Sociais, Rio de Janeiro, v. 54, n. 2, p. 389-430, 2011. Disponível em:<Disponível em:https://www.scielo.br/j/dados/a/7zYMRtbyBf3FqvXMLbz5CQb/?format=pdf&lang=pt >. Acesso em:02/03/2021. [ Links ]
MONTMARQUETTE, Claude; MAHSEREDJIAN, Sophie; HOULE, Rachel. The Determinants of University Dropouts: a Bivariate Probability Model with Sample Selection. Economics of Education Review, v. 20, n. 5, p. 475-484, 2001. [ Links ]
NOGUEIRA, Cláudio Marques; NONATO, Bréscia; RIBEIRO, Gustavo; FLONTINO, Sandra. Promessas e limites: o SiSU e sua implementação na Universidade Federal de Minas Gerais. Educ. rev. [online], vol.33, p.e161036, 2017. <https://doi.org/10.1590/0102-4698161036> [ Links ]
PASCARELLA, Ernest T. Student-faculty informal contact and college outcomes. Review of Educational Research, v. 50, n. 4, p. 545, 1980. [ Links ]
PASCARELLA, Ernest T.; TERENZINI, Patrick T. How college affect students: a third decade of research. San Francisco, CA: Jossey-Bass, 2005. [ Links ]
PERNA, Laura. Studying College Access and Choice: A Proposed Conceptual Model. In: SMART, J.C. (Org.). Higher Education: Handbook of Theory and Research. Dordrecht: Springer Netherlands, 2006, p. 99- 157. [ Links ]
PRATES, Antônio Augusto; COLLARES, Ana Cristina. Desigualdade e expansão do ensino superior na sociedade contemporânea: o caso brasileiro do final do século XX ao princípio do século XXI. Belo Horizonte, Fino Traço, 2014. [ Links ]
RIBEIRO, Carlos A. Desigualdade de oportunidades e resultados educacionais no Brasil. Dados: Revista de Ciências Sociais, Rio de Janeiro, v. 54, p. 41-87, 2011.<https://doi.org/10.1590/S0011-52582011000100002> [ Links ]
RISTOFF, Dilvo. Perfil socioeconômico do estudante de graduação uma análise de dois ciclos completos do ENADE (2004 a 2009). Cadernos do GEA, Rio de Janeiro, n. 4, p. 1-36, jul./dez. 2014. Disponível em:<Disponível em:http://flacso.redelivre.org.br/files/2015/03/Caderno_GEA_N4.pdf >. Acesso em:14/03/2021. [ Links ]
ROKSA, Josipa, GRODSKY, Eric; ARUM, Richard, and GAMORAN, Adam. United States: Changes in Higher Education and Social Stratification. In: SHAVIT, Yossi; ARUM, Richard; GAMORAN, Adam (Orgs.). Stratification in Higher Education: A Comparative Study. Stanford, CA: Stanford University Press, 2007, p. 165-94 . [ Links ]
SACCARO, Alice. FRANÇA, Marco; JACINTO, Paulo A. Fatores Associados à Evasão no Ensino Superior Brasileiro: um estudo de análise de sobrevivência para os cursos das áreas de Ciência, Matemática e Computação e de Engenharia, Produção e Construção em instituições públicas e privadas. Estudos Econômicos. São Paulo, vol.49n.2, p.337-373, abr.-jun. 2019.<https://doi.org/10.1590/0101-41614925amp> [ Links ]
SANTOS, Clarissa; LIMA, Raquel; CARVALHAES, Flavio. O perfil institucional do sistema de ensino superior brasileiro após décadas de expansão. In: BARBOSA, Maria Ligia (Org.). A expansão desigual do ensino superior no Brasil. Curitiba: Appris, 2020, p. 27-57. [ Links ]
SANTOS Junior, José; REAL, Giselle. A evasão na educação superior: o estado da arte das pesquisas no Brasil a partir de 1990. Avaliação: Revista Da Avaliação Da Educação Superior, 22(2), p.385-402, 2017.<https://doi.org/10.1590/S1414-40772017000200007> [ Links ]
SENKEVICS, Adriano Souza; MELLO, Ursula Mattioli. O perfil discente das universidades federais mudou pós-lei de cotas? Cadernos de Pesquisa [online], v. 49, n. 172, pp. 184-208, 2019. <https://doi.org/10.1590/198053145980>. [ Links ]
SENKEVICS, Adriano Souza. “A expansão recente do ensino superior:” Cadernos de Estudos e Pesquisas em Políticas Educacionais 3, no. 4 (April 22, 2021): 48-48. https://doi.org/10.24109/27635139.ceppe.v3i4.4892. [ Links ]
SHAVIT, Yossi; BLOSSFELD, Peter. Persistent inequality: changing Educational Attainmet in Thirteen Countries. Boulder, CO: Westview, 1993. [ Links ]
SILVA FILHO, Roberto; MOTEJUNAS, Paulo; HIPÓLITO, Oscar; LOBO, Maria Beatriz. A evasão do Ensino superior brasileiro. Cadernos de Pesquisa, v.37, n.132, p. 641-659, 2007.<https://doi.org/10.1590/S0100-15742007000300007> [ Links ]
SILVA, Glauco Peres. Análise de evasão no ensino superior: uma proposta de diagnóstico de seus determinantes. Avaliação, v.18, n.2, p. 311-333, jul. 2013. <https://doi.org/10.1590/S1414-40772013000200005> [ Links ]
SINGER, Judith; WILLETT, John. Applied longitudinal data analysis: Modeling change and event occurrence. Oxford University Press, 2003. [ Links ]
SPADY, William G. Dropouts from higher education: toward an empirical model. Interchange, v. 2, n. 3, p. 38-62, 1971. [ Links ]
SUHRE, Cor J.; JASEN, Ellen P.; HARSKAMP, Evert G. Impact of degree program satisfaction on the persistence of college students. Higher Education, v. 54, n. 2, p. 207-226, 2007. [ Links ]
TINTO, Vincent. Dropout from Higher Education: A Theoretical Synthesis of Recent Research. Review of Educational Research, 45(1), p.89-125, 1975.<https://doi.org/10.3102/00346543045001089> [ Links ]
TINTO, Vincent. Leaving college: rethinking the causes and cures of student attrition. University of Chicago Press; 2nd ed. Edição, 2012. [ Links ]
VITELLI, Ricardo; FRITSCH, Rosangela. Evasão Escolar na Educação superior: de que indicador estamos falando? Estudos em Avaliação Educacional, v. 27, n. 66, p. 908-937, 2016. <https://doi.org/10.18222/eae.v27i66.4009> [ Links ]
WOOLLARD, Matthew. Administrative Data: Problems and Benefits. In: DUSA, A.; NELLE, D.; STOCK, G.; WAGNER, G.(Orgs.). Facing the Future: European Research Infrastructures for the Humanities and Social Sciences. Berlin: SCIVERO, 2014, p.49-60. [ Links ]
ZARIFA, David; HANGO, Darcy; PIZARRO, Milian Roger. Proximity, prosperity, and participation: examining access to postsecondary education among youth in Canada’s provincial north. Rural Sociology, v. 83, n. 2, p. 270-314, 2018. [ Links ]
ZWICK, Michael. Stuttgart dropout study 2009. Satisfaction with the course and tendency to drop out among students of the BA course in social sciences at the University of Stuttgart. Stuttgart contributions to risk and sustainability research, n. 14, p. 1-55, 2009. [ Links ]
Received: December 21, 2021; Accepted: July 07, 2022