Introduction
Of all indicators in the Brazillian higher education quality assurance framework, the IDD or “Indicator of the difference between observed and expected outcomes'' is the least well understood. The IDD is the result of so-called value-added modeling, an assessment strategy which aims to take into account student characteristics to isolate the causal effect of schools, programmes or teachers on learning outcomes. Value-added modeling is well known in secondary schools for accountability purposes, but is less used in higher education, in part because the much larger domain of educational outcomes makes it harder to construct valid standardized tests. In Brazil, the IDD indicator has the largest weight (35%) in the composite indicator for the quality of established undergraduate programmes, but it has not been discussed much in the assessment literature. In this paper I discuss the way the IDD is calculated, demonstrate an error in the published values since 2014 and show that this error has policy relevant consequences.
Brazil is one of only a few countries that uses a large-scale standardized assessment system, called ENADE or “National Exam of Student Performance”, to measure the output of its higher education system. In addition, it is the only country that uses the result of this assessment for quality assurance purposes (OECD, 2018). As in other countries in Latin America, the enormous expansion of its HE system in the last twenty years, achieved mainly by private institutions, led to increasing demand for regulation and accountability. The system of regulations put in place, SINAES (“National System of Higher Education Assessment”) relies on quality indicators of inputs of the educational system such as teacher qualifications and infrastructure, but is mainly determined by its outputs as measured by the ENADE assessment of students at the end of their course1 (INEP, 2015). The ENADE exam and collection of other indicators used in the SINAES quality assurance framework is organized by the national educational statistics agency INEP (“National Institute of Educational Studies”). The ENADE exam assesses students at the end of their course, in three year cycles and divided by academic area, each one with its own version of the exam.
There is a large critical literature about the Brazillian higher education assessment framework in general (LACERDA; FERRI; DUARTE, 2016; POLIDORI, 2009), and about its specific indicators in particular (IKUTA, 2016; LACERDA; FERRI, 2017; PRIMI; SILVA; BARTHOLOMEU, 2018). In its conception, the SINAES assessment framework was designed to assess institutions, courses and students in both qualitative and quantitative ways, including institutional self-assessments, a summative assessment of students through the ENADE exam and peer assessments of courses. However, over the years the quantitative aspects of the assessment framework have come to dominate. According to Lacerda, Ferri and Duarte (2016) the accountability function of ENADE has become its most prominent use, in detriment to its function in an assessment system in a more wider sense. One way this happens is through its use in a composite indicator called CPC (“preliminary course classification”), the most important quantitative indicator of the quality of a course in the SINAES regulatory framework. The CPC reduces and quantifies a complex reality into a five category classification (LACERDA; FERRI, 2017). The CPC, which is in actual practice not “preliminary”, uses both the inputs of the educational process like teacher qualifications or infrastructure and outputs such as the ENADE exame, interpreted as a student performance indicator. The largest weight in the CPC indicator is given to the ENADE exam: 20% directly and 35% indirectly through the IDD value-added indicator, which is the focus of this work.
Besides the technical notes of INEP (INEP, 2020a, 2020b), the IDD value-added indicator is not much discussed in the academic literature. It is usually mentioned in passing as one of the indicators in a discussion of the undergraduate course quality assessments (FERNANDES et al., 2009; POLIDORI, 2009), or in an expository fashion (BITTENCOURT et al., 2008). Primi, Silva and Bartholomeu (2018) attempted a value-added analysis of ENADE data from 2006, concluding that it is difficult to construct a value-added measure because most variation of the ENADE scores is within courses, not between courses, especially after controlling for incoming student characteristics. One of the few recent academic works that focuses specifically on the IDD indicator and value-added modeling is a Master thesis (FERNANDES, 2020) that gives a good overview of the context and history of value-added modeling in Brazil and elsewhere. The author compares quantitatively the various models used by INEP and her own similar multilevel model, but appears to use the same, flawed, methodology as INEP to extract value-added measures.
Two recent reports that discussed the quality assurance framework of INEP, are especially relevant. The OECD reviewed the “relevance, effectiveness and efficiency of the external quality assurance procedures'', concluding with respect to the ENADE that its objectives are unrealistic and pointing to weaknesses in its design that ''undermine its ability to generate reliable information on student performance and programme quality” (OECD, 2018). The authors cast doubt on the reliability of CPC and its largest component, the IDD indicator. They discuss qualitatively how the IDD is constructed and the assumptions necessary for its interpretation, but do not make a quantitative analysis. The Brazillian Court of Accounts has done an audit on the quality assessment framework on INEP (TCU, 2018). Among other criticisms, it questions the very notion of added value in this context, maintaining that criterion referenced indicators are more appropriate than the norm referenced methodology in use, which compares courses to each other instead of with reference to content and skills. The audit also calls attention to the arbitrariness of the 35% weight of the IDD indicator.
Outside of Brazil, there have been some studies of value-added modeling in higher education, notably the CLA assessment (STEEDLE, 2012), that uses value-added modeling to report their results. The OECD (2013) has organized a pilot study of a standardized assessment for higher education (AHELO),that included a useful survey of value-added methodologies (KIM; LALANCETTE, 2013). Also notable in this context are statements regarding value-added modeling of the professional associations of statistical and educational researchers, giving guidance on its risks and appropriate use (AERA, 2015; AMERICAN STATISTICAL ASSOCIATION, 2014).
This paper has three main objectives. First, to give a clear exposition of the meaning of the IDD quality indicator, and the way that INEP has calculated it since 2014. Second, to demonstrate how INEP’s calculation is in error and third, that this error is consequential with respect to the reliability of the measure and the classification of courses. In the next section I explain how the IDD indicator is calculated and demonstrate the flaw in INEP’s methodology. Next, I compare the calculated IDD indicator with the corrected methodology, and explore some consequences for the reliability of the measure and the classification of courses. I conclude with a brief discussion.
Value-added analysis and the IDD indicator
Arguably, what matters in quality assessments of schools, teachers or institutions is how much they contribute to the learning of their students. In a quality assurance framework that aims to assess programmes and institutions of higher education, a possible criticism of only using outcomes like student performance indicators would be that those institutions or programmes that take in academically weaker students should be rewarded if they are able to get these students to higher than expected performance levels at the end of the formative period. Value-added analysis in education aims to control the measurement of learning outcomes for the qualities that the students already had and separate them from the contribution of the school or teacher, taking into account somehow the characteristics of incoming students and measuring the performance of concluding students in relation to expectations. Value-added analysis is a kind of growth modeling with the extra requirement that the result speaks to the impact of the institution on the students growth, above and beyond a mere description of growth (OECD, 2013). >are many kinds of growth models (CASTELLANO; HO, 2013). Since 2008, INEP has made use of variations of residual gain models or covariate adjustment models to calculate its IDD value-added indicator. These models model the outcome, in INEP's case the ENADE score, by regressing it on variables that depend on incoming student characteristics. The model leads to a prediction of what the ENADE score should be, given student characteristics. The difference (residual, in regression terminology) with the actual ENADE score is then a proxy for value added.
There are some essential assumptions that must be made if we are to believe in the validity of the value-added measure obtained in this way (OECD, 2018) to assess courses. First, the assumption that it is possible to consider the indicators chosen to measure incoming student performance as good predictors for academic performance in higher education. Second, that the ENADE score itself is a valid and reliable indicator for academic outcomes. And third, that the difference between expected and observed outcomes are really due to the characteristics of the institution and not to some other variables that have not been taken into account by the model.
The original SINAES legislation from 2004 actually stipulated that both incoming as well as concluding students should be tested, so that the performance of incoming students could be used to estimate expectations for concluding students. Since 2012, however, the ENADE exam has been taken only by concluding students. The national higher education admission exam ENEM (“National Exam of Secondary Education”) took the role of proxy for the characteristics of incoming students. As before, in 2012 and 2013 regression analyses were made using the course averages of ENADE, ENEM and other variables. A consequential change was made again in 2014, when instead of a regression of averages, INEP started using a regression model of individual student ENADE scores against their ENEM scores from some years ago. This was made possible because by 2014 enough concluding students could be identified in the ENEM database (which had started its function as an admission exam to higher education in 2009).
This new IDD calculation in place since 2014 uses a multilevel model (or HLM, hierarchical linear model) to model the ENADE score of individual students as a function of their scores on the ENEM admission exam. In education research, multilevel models are used because they are statistically more efficient and reliable compared to models that use aggregated scores since they explicitly take into account the fact that students are clustered within institutions (or, as in the case of the ENADE assessment, courses). In principle, multilevel modeling would also enable the use of both student characteristics and course and/or institution characteristics to predict concluding student performance. In the case of the IDD as calculated by INEP since 2014 however, the expected value of the ENADE score of concluding students of a course is predicted solely by their scores on the ENEM admission exam a few years earlier.
For expository reasons, I first treat a simplified version of the model, with only one covariate, that is easier to explain and visualize. The statistical specification is
(1)
(2)
(3)
The first, student level, equation is just the assumption that the ENADE score of a student i in course c can be modeled as a linear function of the students ENEM score, with intercept ß 0c and slope ß (in the real model used by INEP the four subscores of the ENEM exam are used as covariates). The errors ϵ ic represents the random measurement error of the performance of student i in course c which are taken to be normally distributed and independent of the ENEM covariate, per the usual regression assumptions. The second equation is on the course level and expresses that the intercepts on the student level are composed of an overall mean intercept for all courses ß 00, plus a value u c for each individual course. The value u c will be estimated from the data and will be identified with the added value of a course, or at least its contribution to its students ENADE score, given their ENEM scores. As an illustration, simulated data is shown in Fig. 1a (left) for four hypothetical courses, constructed to have students with different average ENEM scores and which confer different value-added. The ENADE scores of all students, on average, follow Eq. 1 with a constant slope, but Course 1 was able to add 10 points compared to all other courses and Course 2 subtracted 5 points. It is clear that the intercepts compared to baseline, 10 and -5 in this case, should be identified with the “added value” of the course, when differences in the average ENEM score of students are taken into account.
Fig. 1b (right) shows actual data from two courses in the Civil Engineering academic area that illustrates two points. First, it’s clear that the ENADE score is indeed related to the ENEM score of students, as expected, although the assumptions of the model should still be subjected to formal statistical verifications, something that is out of scope for this paper. Second, most variation of the ENADE scores of students is within courses, not between courses, something also observed by Primi, Silva and Bartholomeu (2018). This means it will be difficult to construct value-added measures with precision or reliability. Note that the two courses shown in Fig. 1b (right) were chosen to be extremes for expository reasons, and that all other courses have lower estimated intercepts (the estimated value-added or IDDs).
In hierarchical models like these, the varying intercepts u
c
that represent the value added by a course can be estimated in two ways from the data. One way is to treat the effect u
c
of a course on the outcome score (the ENADE score, in our case) as a so-called fixed effect. In this case, the effect, or intercepts, u
c
are estimated only from student data within each course. In contrast, in a so-called random effect model, which is what INEP uses for their IDD calculations, the intercepts are assumed to be sampled from a normal distribution with a standard deviation
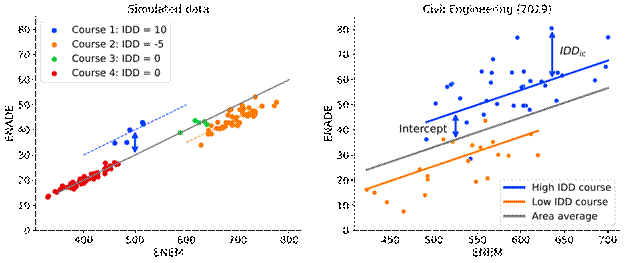
Source: 1a (left) simulated data, 1b (right) calculations by the author and microdata from INEP (2020c). Note: Fig. 1a (left): simulated data. Fig 1b (right): student data from two courses in the Civil Engineering area, obtained from the 2019 IDD microdata, with fits from a multilevel model. One estimated intercept and a student residual as used by INEP ( IDD 𝑖𝑐 ) to obtain course IDDs are so indicated.
Figure 1 Simplified multilevel model for value-added modeling with only one dependent variable, the total ENEM score. Each point is a student and the lines represent the model fit.
We can now state again the main claim of our paper, which is that since 2014, INEP has calculated its IDDs incorrectly. Instead of using the estimated intercepts of the multilevel model (indicated as Intercept in Fig 1b), INEP takes the average student residual with respect to the whole model (indicated as IDD ic in Fig 1b). In other words, INEP takes the average of the residuals of students ENADE scores with respect to the individual course regression lines (the upward and downward shifted lines in Fig. 1 that best predict ENADE scores of a course given student ENEM scores). One of those residuals is indicated as in Fig 1b (right). It should be clear that this is not the correct procedure. We support our claim first by simply pointing to the oficial INEP documentation and then also by reproducing exactly the incorrect INEP IDDs, using the microdata and the incorrect procedure.
In the “Technical Notes” that INEP publishes, the explanation of how the IDD is calculated has not changed since the 2014 edition. In the following we quote from the “NOTA TÉCNICA Nº34/2020/CGCQES/DAES” published in 2020 and referring to the 2019 edition of the ENADE exame and IDD calculation (INEP, 2020b). In this official documentation (the “INEP note” in what follows) the procedure to calculate IDD scores of a course in a certain academic area is detailed. The raw IDD score (before normalization and rescaling) is defined as the average of all participating students' individual IDD scores (equation (7) from the INEP note). In turn, equation (6) defines these student IDD scores as2
where C ic is the observed ENADE score of student i in course c. Equation (5) of the INEP note defines
with
with the explanation that u oc “...is the random effect associated with the course c”. The INEP note makes clear that they are estimating a linear multilevel regression model with fixed and random effects. The equations of the INEP note are equivalent to our simplified model specified in equations (1) and (2) (except for using the four ENEM subscores). However, the INEP note does not identify the course residual u oc with the course value added, which is the natural procedure adopted in the academic literature (see Fig. 1a and the equations in Kim and Lalancette (2013).
Instead, INEP calculated the course IDD from the averages of student residuals IDD ic . But the crucial detail to note in the equations from the INEP note is the inclusion of u oc in the calculation of this student residual. According to INEP the student residual IDD ic is the difference between the observed ENADE score and the course regression line, instead of the academic area average regression line (see Fig. 1b). This erroneous procedure (partially, as we shall see) defeats the purpose of a value-added measurement, by averaging out exactly the part of the ENADE score that the course contributed to. The next section will discuss how the precise details of the estimation method for the course residual u oc prevents the course IDD as calculated by INEP, being a sum of residuals around u oc , to just produce a mean of zero with a random error term.
Further evidence that this interpretation of the INEP note is correct, is the fact that it is possible to reproduce3 exactly the published course IDD values using the erroneous procedure, the published microdata from the 2019 assessment cycle (INEP, 2020c) and open source software (BATES et al., 2015, p. 4; JOLLY, 2018). For example, my calculations coincided with INEP’s to within 0.01 points on the ENADE scale for 231 of the 232 courses in the Medicine academic area (keeping in mind that the raw IDD have a typical range of 10 points on the ENADE scale). In every academic area of the 2019 cycle, there were typically only between 1 or 5 courses (less than a few percent of courses) with INEP IDD scores that we could not reproduce exactly (maybe due to a different treatment of outliers).
In the next section comparisons will be made between the INEP method of averaging student residuals and the “Intercept” method that simply identifies the varying intercept u c of Eq. (2) with the IDD value-added indicator for each course. The very precise reproduction of the published IDD scores with the INEP method gives confidence that the course intercepts u c were calculated correctly. The only difference between the INEP method and the Intercept method for the determination of the IDD value is the correct use of the varying intercept multilevel model, with the model specification, the student data and the estimation methods all being the same as INEP used in their calculations.
Comparisons and consequences
The mistake identified above for how the IDD is calculated has real consequences, for the classification of courses and also for the reliability of the IDD indicator. Before discussing these consequences, however, it should be clear that the two methods are not independent. In fact, the (Pearson) correlation between the two methods across the 29 academic areas that were assessed in 2019 is between 0.6 and 0.7. Figure 2 shows a visual comparison between the IDDs of courses calculated with the INEP method and those calculated with the Intercept method for four academic areas.
The question arises, if the INEP method really takes the average of residuals, why does it not produce meaningless noise around a mean of zero? And why would there be a correlation between the two IDD calculation methods? The answer is the particular estimation method of the linear mixed model used, which biases the course intercepts u oc towards the academic area mean. This so-called shrinkage is not necessarily a feature of the statistical model as specified by Equations (1) - (3). Rather, it is a feature of the specific way how the course intercepts are estimated. The shrinkage effect can clearly be seen in Fig. 1b: the student residuals are not spread symmetrically around the estimated course intercepts, which are “shrunken” towards the avea average. The resulting asymmetry of the student residuals around the intercept leads to correlation between the Intercept and the INEP method.
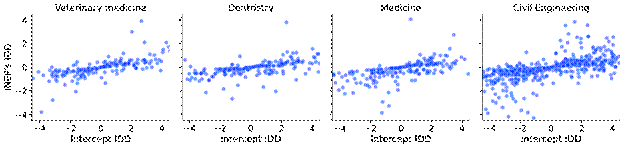
Source: calculations by the author, using microdata from INEP (2020c). Note: A direct comparison between the raw IDD values (on the ENADE scale, before normalization and rescaling) of courses calculated according to the INEP method and the Intercept method.
Figure 2 Comparison between INEP and Intercept IDD values
The correlation between the two methods notwithstanding, there are nonetheless real and policy relevant differences. We focus first on the categorical classification of courses imposed by INEP into “satisfactory” and “unsatisfactory” categories and later on the reliability, a measure of the internal consistency of the indicators.
As with their other indicators that are part of the SINAES quality assurance framework, INEP communicates the raw IDD indicator, a number on the ENADE scale, by normalizing and rescaling to a discontinuous 1 through 5 categorical scale (outliers with more than three standard deviations are mapped onto the 1 and 5 categories). In communications with the public it is made clear that the middle category (3) is considered “satisfactory”. In the case of the CPC indicator (in which the IDD indicator has a weight of 35%, see above) the categories 1 and 2 lead to real consequences in that they are considered insufficient and give rise to an “in loco” visit for re-credentiation purposes. It makes sense therefore to investigate how many courses move from a 1 or 2 category (unsatisfactory) to a 3 or higher one (satisfactory) and vice versa. We found that in for example the Medicine academic area of 232 courses assessed in 2019, 9 courses went from a 1 or 2 category (unsatisfactory) as calculated by the INEP method to a satisfactory (3 or above) category, whereas 33 courses went from a satisfactory or higher category to an unsatisfactory classification. As another striking example, in the Civil Engineering area, 231 courses (32% of the total of 715 courses) went from a satisfactory category to a below-satisfactory category. Table 1 summarizes these numbers for a few academic areas (the numbers for other areas lead to the same conclusions).
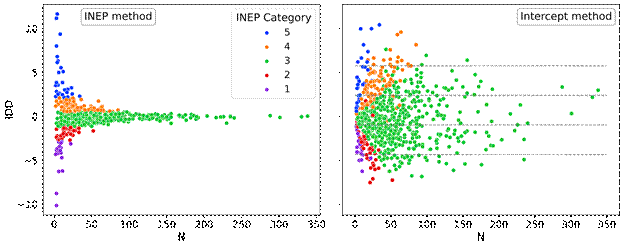
Source: calculations by the author, using microdata from INEP (2020c). Note: Raw IDD scores of courses in the Civil Engineering academic area, as a function of the number of students per course taken into account in the calculation. Fig 3a (left): INEP method. Fig 3b (right): Intercept method. Note the typical funnel shape for the INEP method (left) and how a large number of courses in the green “3” or “satisfactory” category end up in the unsatisfactory 1 e 2 categories with the Intercept calculation. Colors indicate the category as assigned by INEP and the horizontal lines in the right figure are the boundaries of the categories using the same methodology applied to the IDD calculated with the Intercept method
Figure 3 IDD values of Civil Engineering courses (2019)
Figure 3 shows the comparison between raw IDD scores for all courses in the Civil Engineering academic area, calculated according to the INEP and the Intercept methods, as a function of the number of students in the course. The INEP method (Fig. 3a) shows the typical funnel shape characteristic of the means of (semi-)random numbers which indicates low reliability of the INEP method. As is clear from the graph, essentially all courses in the highest and lowest categories are those with fewer than 50 students or so. The IDDs calculated with the Intercept method, on the other hand, do not show such a pronounced funnel shape, indicating a higher reliability. It is also clear that for many courses the new IDDs would be assigned a different category, as indicated by the category boundaries (which are converted to the raw IDD scale using the same normalization and rescaling methodology that INEP uses).
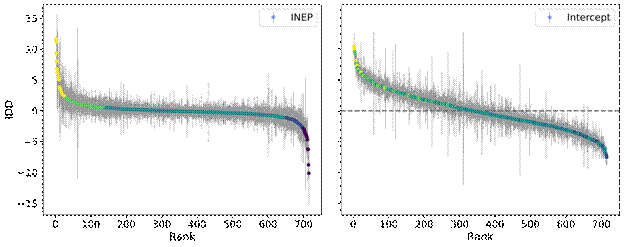
Source: calculations by the author, using microdata from INEP (2020c).
Figure 4 Same data as in Fig. 3, but with courses ordered by rank and an estimate of the standard error
In Figure 4 we again plot the IDDs calculated with the two methods side by side, but now ordered by rank. For every course IDD we also visualize the standard error, estimated for the INEP IDD simply by the standard deviation of the residuals divided by the square root of the number of students in the course (the same standard errors are used for the Intercept method). Again, the conclusion that can be drawn is that the Intercept method is more reliable than the INEP model, since the highest and lowest ranking courses differ by a few standard errors for the Intercept method, but they don't for the INEP method, making the ranking unreliable in the latter case.
Table 1 Comparison of the INEP calculations of the IDD indicator (that we reproduced using the 2019 microdata) and the Intercept method, for selected academic areas
Academic Area | Courses | Reliability | Course classification changes (INEP vs Intercept method) | ||
---|---|---|---|---|---|
N | INEP method | Intercept method | unsatisfactory to satisfactory | satisfactory to unsatisfactory | |
Veterinary Medicine | 214 | 0.60 (0.01) | 0.822 (0.002) | 18 (8%) | 16 (7%) |
Dentistry | 237 | 0.65 (0.01) | 0.880 (0.001) | 25 (11%) | 9 (4%) |
Medicine | 232 | 0.74 (0.01) | 0.851 (0.002) | 9 (4%) | 33 (14%) |
Civil Engineering | 715 | 0.703 (0.005) | 0.834 (0.001) | 4 (0.6%) | 231 (32%) |
Source: calculations by the author, using microdata from INEP (2020c).
Note: The reliability estimates were made with the split sample method (repeated 50 times, with the standard error in parentheses). “Unsatisfactory” and “satisfactory” refer to being in the “1” and “2” category and “3” or above respectively, according to INEP’s method of normalizing and rescaling the raw IDD scores into five categories. The number of classification changes from the INEP method to the Intercept method is indicated. For example, 33 of 232 courses (14%) in the Medicine area would be classified as unsatisfactory if the Intercept method were used.
The improvement of the internal consistency of the Intercept IDD indicator alluded to above can be quantified by comparing the so-called split sample reliability for the two methods. Conceptually, the reliability of a measure is the correlation between “parallel tests” or repeated applications of the instrument. However, the usual reliability indicators like Cronbach’s alpha are not so easy to calculate in this case. Following Steedle (2012), for each course I determined two new IDDs, each calculated with half of the students taken randomly from the course. These two IDDs are then like parallel tests and the correlation between them can be considered an estimate of the reliability of the indicator. The values for the Pearson correlation reported in Table 1 are corrected by the Spearman-Brown factor (2r/(1+r), with r the half sample correlation, to take into account the fact that only half of the sample was used in each calculation. These split sample reliabilities were calculated 50 times, to get an idea of the sample standard deviation.
The results are shown in Table 1, and it’s clear that the Intercept method has a significantly higher reliability than the INEP method, elevating this measure of reliability from 0.6-0.7 to above 0.85. The latter are comparable to the split sample reliabilities of around 0.75 obtained by Steedle (2012) for the CLA assessment (which also uses a multilevel model to obtain a value-added estimate).
Discussion
I have argued that INEP since 2014 has miscalculated the IDD value-added indicator of its undergraduate programme quality assurance framework by incorrectly using their statistical multilevel model. The consequences of this mistake are a significant decrease of reliability in a statistical sense and the existence of a large number of “satisfactory” courses that should have been classified as “unsatisfactory”, and vice-versa, according to INEPs own standards.
If INEPs multilevel model is used for value-added calculations, then it’s necessary to identify the varying intercepts with the IDD. However, even if the correct method is used, it is not clear what the reliability or validity of the corrected value-added measures are. The statistical model, the outcome and predictor variables chosen, all of these surely are subject to all kinds of limitations that should be evaluated critically. This paper only compared and contrasted an incorrect method with a correct one, using the exact same model and data. There may be better models to calculate value-added indicators for Brazillian higher education undergraduate courses, but the large within-course variability compared to the between-course variability of the ENADE scores will always limit their reliability.
What could explain the incorrect calculation of the IDD by an organization known for its competence in educational statistics? The fact that the only courses in the highest or lowest categories were those with a small number of students participating should have raised the alarm, since that phenomenon is typical for indicators with a large random component (Fig. 3a). Before 2014, the IDD was calculated by a residual gain model using the average ENADE scores of courses, in which case the added value is indeed the residual with respect to this model. It may have felt natural to assume that a more sophisticated model using individual student scores like the multilevel model would also use residuals in some way. Without visualizations like those of Figure 1, the multilevel model is not so easy to interpret just looking at the equations.
One conclusion to be drawn from this episode is that the opaqueness of how a performance indicator is constructed is a real problem. During six years and two cycles of measurement the publication of an incorrectly calculated and unreliable performance indicator did not once lead to objections of the interested parties like higher education institutions or the public in general. Given this fact, it is hard to make the argument that the indicator is performing its accountability role properly. Others authors have already called attention to the fact that the INEP quality assurance effort is low stakes for public institutions, which rely on other factors to attract students but high stakes for private institutions (TCU, 2018). That some indicators behave like a weighted random number generator is maybe not so bad for some stakeholders, since there is a chance to promote the one that in a particular year turned out to reflect well on them, and this may further explain the lack of pushback.
The challenges of designing any large scale standardized assessment are enormous, as the large scholarly literature about them makes clear. These challenges are exacerbated in higher education, where the assessed domains are much larger and correspondingly harder to measure even in areas where there is consensus about learning outcomes (KORETZ, 2019). There have been few studies that assess the validity and reliability of the ENADE assessment and INEP does not report indicators of precision or reliability of the scores. The IDD is a value-added measure that derives from the ENADE score, and this further modeling introduces even more uncertainty. The results reported here are therefore not surprising and in line with the general tenor of the criticism of the OECD committee which concluded that the ENADE assessment is not fit for purpose, or at least not for all the purposes that are being asked of it.
A problem with assessments like ENADE is that they are used for many things at the same time (for both monitoring of quality and institutional accountability, for example) and that the inferences made from its scores are too broad (KORETZ, 2019). One lesson to be learned is that stakeholders should demand validity and reliability studies of the indicators to which they are subjected (AERA; APA; NCME, 2014). Additionally, to improve how the quality assurance indicators are used by administrators and the public in general, INEP should find a way to better communicate the uncertainties in their measures and estimates of their precision and reliability.
The present study only used the published IDD indicators and the microdata for 2019. The same methodological flaw exists for the calculation of the IDD indicators for all years after 2014. Some open questions that merit further study include test-retest reliabilities (longitudinal studies comparing courses over assessment cycles), comparisons with the value-added methodologies used before 2014 as well as a broader investigation as to the validity and reliability of value-added indicators using ENADE results.